Enterprises that grew up in a relational database world for business intelligence are trying to adapt that mindset to enterprise AI – and are stunting its potential, according to EYC3 managing partner Conrad Bates.
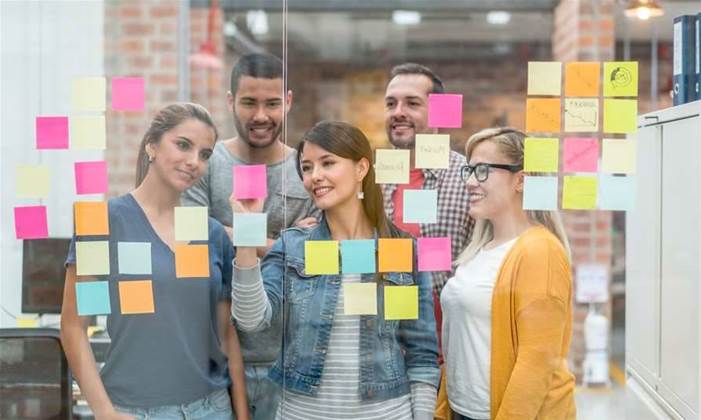
EYC3 is Ernst & Young’s advanced analytics business in Asia Pacific. It is the result of the 2015 acquisition of C3 Business Solutions, which itself is part of a half billion-dollar play by EY to build capability in advanced analytics globally.
“There are many types of advanced analytics,” Bates told iTnews.
“We see AI as one of the classifications of advanced analytics. Sitting alongside that are neural networks and a couple of others.
“Within AI, I see cognitive as a stream of AI in that it’s an AI approach in general on how things are brought together.”
Bates sees a number of opportunities emerging for the new breed of enterprise AI techniques but also some challenges.
One of those is to get clients to think differently about advanced analytics, which Bates says starts with leaving the “relational-based thinking” from previous BI and data warehouse deployments at the door.
“One of the biggest problems that we see at the moment is people who have grown up in the relational database modelling paradigm for a traditional BI and data warehousing domain take relational based thinking into these [new] problems,” he said.
“The reason databases were designed through the 70s, 80s, and 90s, and organised in a relational construct was so that people could write a query and ask the database a question.
“Now, the problem we see many customers doing is when they go to cognitive, AI or advanced analytics solutions, they try and enforce relational-based thinking on the problem.
“If you take relational thinking into an advanced analytics problem, we invariably see clients fail simply because there’s a very different way in which you store and manage data in advanced analytics compared to a relational domain.”
Bates sees EYC3 as a potential bridge between old BI and newer advanced analytics.
“Clients are increasingly asking more complex questions that require more advanced analytics techniques to solve them, yet their only using toolsets that are in the traditional relational world,” he said.
“We have to reorganise the [relational] database to enable advanced analytics techniques.
“We’re invariably bringing more of the new thinking to the client to help resolve the specific analytics questions they’re trying to ask and the scenarios they’re having problems with.”
Not organising data for advanced analytics can have flow-on effects, something Bates said he typically sees from early adopters that come to EYC3 for advice on fine-tuning the accuracy of their chosen algorithms.
“What we would generally find is if you don’t organise data in a way that the machine can maximise its compute advantage, and if you don’t have generally good quality data, then you’re doing yourself a disservice,” he said.
“People will come to us and say, ‘we’re trying to tune our algorithm to increase our resolution on prediction from 85 to 90 percent, what can you do to help us?’
“I’ll invariably recommend adding one or two data elements to an algorithm because it will give you a significant step in increasing your prediction resolution.”
Three targets for enterprise AI
Bates sees three broad areas that are the most conducive to enterprise AI or cognitive projects.
“The ability to grow an organisation’s top-line, optimise a cost or expense problem, and then protect an organisation,” he said.
“Broadly, we’re seeing a lot of cognitive problems currently being applied to the optimise path.
“People are looking at how do I make this process leaner? What are the things I’ve got to do to coordinate this activity that are going to take cost out or improve a process?”
One reason optimisation is being favoured is because these types of problems are typically well-defined and structured, Bates said.
“When you have a cognitive approach, the whole process is a mechanism of identifying a problem, training the computer to do a course of action, and then getting an outcome as a result of it,” he said.
“And in AI, particularly when you think about robotic [process automation], we see well-structured problems that are automated or subject to a high degree of automation pulling together a desired outcome.”
In other words, some advanced analytics techniques work well with structured problems. “Therefore, because the expense side or optimise side of the business is generally well known or well structured, clients seem to spend more time [on AI] there.”
Growing revenue or income is where the problems become less structured, and therefore perhaps less likely to be conducive to resolution through AI.
“In essence you find you’re trying to bridge holes in your data assets to understand behavioural characteristics of, say, consumers or supply in a marketplace,” Bates said.
“They’re less likely to be structured problems, and therefore we find traditional methods of advanced analytics like linear regression or correlation or those types of statistical methods generally apply [better] because you’ve got sample subsets of data that you’re using those techniques to try and resolve.”
Find out how rivals KPMG, Deloitte and PwC are using AI and cognitive computing technologies