Australian physicists are adopting new techniques in machine learning and pushing the limits of high-performance computing in a bid to gain new insights from the CERN Large Hadron Collider in Europe.
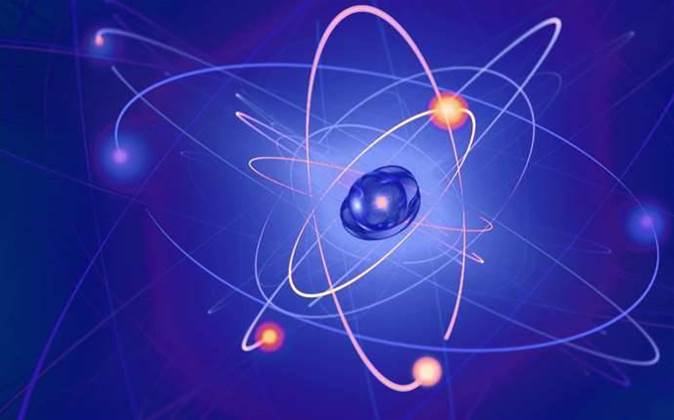
The ARC Centre of Excellence for Particle Physics at the Terascale (CoEPP) is a collaboration between physicists from the University of Melbourne, University of Sydney, University of Adelaide, and Monash University.
It is made up of both experimentalists who generate data and theorists who explain data, and aims to analyse information from the ATLAS experiment at the Large Hadron Collider, as well as other high-energy physics experiments such as Belle II in Japan.
University of Melbourne associate physics professor Martin Sevior told iTnews the researchers confront the physics equivalent of finding a needle in a haystack.
“If you look in multi-dimensional space there’d be a particular region that shows up as Higgs particles with some background. So what we do then is acquire more and more data so see a significant signal above that background,” Sevior said.
“However, the more background you have, the more data you need to see a significant signal. That’s what we did for the initial discovery of the Higgs.”
The researchers' ambitions have grown as their experiments have progressed, leading to an increasing reliance on machine learning techniques and high-performance computing capabilities.
One CoEPP researcher, Noel Dawe, has a machine learning application at the proof-of-concept stage and is testing it to see if it’s suitable for mainstream use in ATLAS.
“What we’ve tried to do is find rarer processes of the Higgs, and finding those means an order of magnitude or two more in background in terms of signal. So to cut that away we have to understand exactly what our background looks like,” Sevior said.
“The way we do that is we look at a particular region in multi-dimensional space where we have no signal but we understand what our background is, and then we train a neural network or a boosted position tree to recognise signal as opposed to background.
“What we’re trying to do is see if the deep learning technologies and multi-layer neural networks can be used to give us even more background rejection.”
Heavy-duty compute capabilities
Storing and crunching the vast quantities of data coming off the Large Hadron Collider and other experiments requires a lot of compute and storage firepower.
All countries that are participating in the ATLAS project, including Australia, are obligated to contribute to the global computing grid used to crunch data from the experiments.
According to Sevior, CoEPP currently runs two main compute clusters, with one dedicated to the grid and the other used for different research purposes, such as comparing data from the LHC to the results predicted through theoretical physics.
The first cluster is dedicated to the ATLAS grid and consists of 57 nodes, providing around 8 teraflops of compute power. The second cluster, for CoEPP’s local researchers’ work, has around 80 nodes and approximately 7.5 teraflops of compute capability.
However, a number of the nodes on the research cluster are currently being reallocated to ATLAS, so CoEPP’s current total contribution to the global grid stands at 91 nodes and 1816 CPU cores. This equates to just over 2 percent of the total ATLAS global grid.
“So the data comes out of CERN, it goes to computers all around the world, we analyse that data and either provide the results to whoever asked for it, or use the data locally because we use the facilities here for our own interesting data analysis,” Sevior said.
“The problem of efficiently moving large volumes of data securely from cluster to cluster is what the grid was designed to do, and it enables ATLAS to work. Without the grid, we’d have tonnes of data from the LHC, but we wouldn’t have found the Higgs particle yet because we’d still be processing data from 2012.”
CoEPP’s contribution to the ATLAS grid is calculated on an assumption that each participant will spend a fixed amount each year on hardware, including upgrades and the replacement of outdated equipment.
Taking into account technology improvements in processing power and storage capability, CoEPP anticipates a 15 percent increase in disk capacity and 20 percent boost to its CPU capacity each year.
Based on this, the centre anticipates it will deliver five times the current CPU performance as it currently does in 2024.
“The CERN grid will continue to grow as the data rates from the LHC increases. There’s a projected increase in LHC intensity from now to 2030 by a factor by 10 to 100. To analyse that, we’ll have to grow our compute resources by a commensurate amount.”
In addition to the two main HPC clusters, CoEPP has a 700 core Nectar allocation that dynamically flows between ATLAS, Belle II and local researcher needs.
“We also use Nectar for the final phase of data analysis. There’s a cluster of computers we’ve set up as a batch system. What we do in particle physics is quite exquisite data science, and one of the things we need to know is where our uncertainties are,” Sevior said.
“One of the ways we do that is through what are known as Toy Monte Carlo experiments, where we generate data that looks like our final plots, and then we fit those data samples and we see how those fits resemble what we put in our model to begin with.
“We test the fitting of our model and understand how well our fits perform on real data. Doing that sort of study takes a substantial compute resource, and that’s provided by Nectar.”
The systems run Scientific Linux 6, along with a range of bespoke pieces of software written in Python and C++, as well as specialist packages such as ROOT..
“[ROOT] does all sorts of sophisticated things like multi-variate analysis, multi-dimensional fitting, it’s robust and extremely well understood," Sevior said.
“Now we’re getting into machine learning, Google’s TensorFlow is something we’re interested in, and for the GPU stuff we use NVidia CUDA.”
Big data storage for quantum particles
The growing need for research compute firepower has been matched by an increase in CoEPP’s data storage needs.
CoEPP currently has 1.1 petabytes of storage for the ATLAS grid, provided using DPM (Disk Pool Manager), of which 300 terabytes is obtained through research computing partnership VicNode.
“The difference between what we do in high-energy physics and what HPC does at, say, ANU is we’re focused on very high data throughput," Sevior said.
“So not only do we have HPC clusters, but we also have very high-performance file systems. And this enables us to pull data out of the file system very fast and analyse it quickly.
“We use commodity hardware because it’s cheap, both in terms of CPUs and disks to get the best bang for our buck, and it’s tied together with the computing middleware that enables the grid to work.”
As a result of its growing storage needs, CoEPP recently added 384TB in raw storage as part of VicNode’s rollout of the Southern hemisphere’s largest usable largest Ceph storage cluster.
Limits of grid computing
Having worked on one of the world’s largest examples of a grid computing deployment, Sevior points out there are numerous limitations to the model.
“What we’ve found that the hurdle for what is known as a grid certificate is quite high. We can require our PhD students to go through that learning curve, but it’s hard to get people who don’t have that commitment to do that,” he said.
“I’m not sure what the long-term future of grid technology will be, certainly we’ll continue to use it, but we’re also interested in other solutions as well. For example, we use cloud technology a lot already, and it could be that a large proportion of our clusters in the future will be used for cloud.”
Sevior describes grid computing as a perfect example of the Gartner hype cycle in action.
“When we jumped into this field in 2001 and 2002, there were lots of people hyping how this is a wonderful new thing that will even slice and butter your bread. Then we hit the peak of the hype cycle,” Sevior said.
“Then it went through a dip when people realised it was hard to use. Now we’re coming out the other side, where we’re actually using grid technology. It’s a fascinating example of human nature at work.”
New Spartan GPU nodes
With an increasing interest in machine learning and neural networks, CoEPP has recently begun adding GPU nodes to its supercomputing armoury.
The organisation has agreed to co-invest in a GPU expansion of University of Melbourne’s new Spartan HPC cluster, which was officially launched last month.
The centre will buy two dedicated nodes with two Nvidia K80 GPU cards, while the university’s IT services will purchase an additional server, bringing the total number of GPU nodes on the HPC system to five.
“We’re also using GPU technology to test using deep learning networks because a lot of what we do is looking for rare and subtle effects. We’re using deep learning to see if we can improve our ability to distinguish between signal and background,” Sevior said.
“Because what we try to do in particle physics experiments is dig out very small signals against a very large background. For example, it takes well over a trillion proton interactions to see just one Higgs particle.
“Our initial tests were on a GPU system that was loaned to us by the UoM, but we’ve decided to buy our own because our tests were so successful.”