BMW is turning to machine learning to help its dealers diagnose defects with cars that are brought in for servicing under warranty.
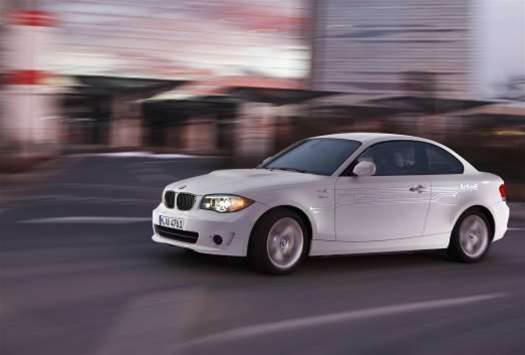
Engineer Marc Kaminski told the Spark Summit Europe late last month that the carmaker is using the large amount of data generated by its vehicles to recognise problems that would otherwise be difficult to manually diagnose.
Kaminski and his team are trying to lower the number of “no trouble found” cases seen by dealers’ workshops, where a car is brought in under warranty to be fixed but no specific problem is found.
“Not finding the correct defect is a huge cost factor for car manufacturers. In fact, according to a study of consulting firm BearingPoint, it is the number three contributor for warranty incidents,” Kaminski said.
“No trouble found cases are cases where the customer brings their car to a dealership and they don’t really know what the real problem is or they know the problem but not the root cause.”
Dealers’ workshops typically rely on the skills of their team as well as manual processes to try to work out what might be causing the owner of a returned car grief.
“The [process] we use today for finding steps for doing diagnostics on cars - as well as the diagnostics itself - are quite manual,” Kaminski said.
“But that manual approach just cannot handle the vast number of possibilities that are present in a car anymore, if ever.
“That is strengthened by the fact that cars are getting more complex due to hybridisation where we have several engines in one car and the connectivity between the sensors in the car and all the electrical parts.”
The combination meant customers could be sent away with an ineffective fix, causing dissatisfaction down the road when they “might have to return for the same defect again".
Kaminski saw an opportunity to solve the problems with a data-driven approach, particularly given the large amount of data being generated by BMW vehicles.
“We just want to take the data that is in the car already, take that data from many cars, build models and then use those models for predicting the defect in the dealership directly so the workshop staff can fix the car immediately,” he said.
The carmaker built a dataset that contains all defects that can occur in some of its models, and split the data “into different categories of cars which share the same defect”.
The dataset is unsurprisingly complex: it captures an enormous amount of information from cars, but the amount of data capture varies from field-to-field, and the dataset as a whole “is quite skewed - defects only occur in a very small amount of cars if you look at the whole fleet”, Kaminski said.
“So those three challenges we wanted to solve in our machine learning pipeline,” he said.
“We went with Apache Spark for doing the whole pipeline because it gives you an opportunity to do all those steps in one framework.”
Kaminski said Apache won out over other options because some frameworks couldn’t keep up with the size of the dataset.
“We wanted the pipeline to be future proof because we expect the dataset to grow [in future],” he said.
The carmaker is using the machine learning models in part to understand what bits of data its vehicles capture could lead to a better diagnosis of a defect.
“We can use that knowledge to think about the design of our cars or solve some liability issues with subcontractors,” Kaminski said.
The defect diagnosis project is also expected to be a stepping stone to predictive defect diagnosis in future, where BMW may be able to recognise in real-time the onset of an issue.
“This is the first building block for building predictive maintenance which means we can tell the customer while they’re driving that they should go to a workshop soon,” he said.
Download your copy of iTnews' report on the adoption of machine learning and artificial intelligence within Australian enterprise.