The team from the Warmest 100 are back with what they’re calling “the web’s most accurate prediction of Triple J’s Hottest 100”, with a swag of new tricks up their sleeve.
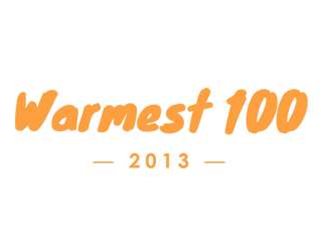
Last year, a group of data analysts successfully predicted 92 of the 100 songs in the world's largest music vote (though mostly not in order) by mining data from posts on social networks auto-generated by the broadcaster to further expand the reach of the massive voting exercise.
So accurate were their predictions that this year, Triple J disabled the social sharing function that allowed for the data to be scraped.
Yesterday, The Vine reported that the team, who initially weren’t planning a repeat attempt, had a change of heart on Sunday after encouragement from Chicago economist David Quach, and have compiled a new list for this year.
Today iTnews went behind the scenes to find out how they did it.
On Sunday morning, Australia time, Quach contacted Nick Drewe from last year’s Warmest 100 team to say that he’d collected around 400 votes from a search of Twitter, and asked Drewe if he was sure he didn’t want to run the Warmest 100 again.
People had been posting images showing their votes, he noted, and Quach had manually read them and tallied them up. Drewe had a change of heart after repeating Quach’s method.
Instagram “turned out to be a goldmine”, and Drewe re-used code from an Instagram search tool he’d written to search for images tagged with “hottest100”. His code used the Instagram API to find the images, and simply downloaded them.
The team then used a free trial of Optical Character Recognition (OCR) software called Maestro to process the images and extract the votes. The votes were tallied in a simple spreadsheet.
Independently, Mark Pazolli, an engineer and mathematician from Western Australia, developed his own, similar method to that of the Warmest 100 group. He decided to try after hearing that the Warmest 100 wouldn’t run again.
“When I heard the guys weren’t doing the Warmest 100 again, I thought, ‘why not?’” he said.
Pazolli’s more sophisticated approach allowed him to complete his own list ahead of the Warmest 100 team, as they publicly acknowledged via Twitter.
Pazolli also used the Instagram API to find the source images containing people’s votes, using a program he wrote in Python. He then used wget to download the images, and the open-source OCR program Tesseract to process the images.
Some more Python code cleaned up the resulting text file, which was cross-matched with a list of artists and song titles provided in a pdf by TripleJ to all Hottest 100 voters, again using Python.
Pazolli tried various matching methods, eventually using a locally-sensitive hash called a Nilsimsa hash augmented with some hinting to offset the method’s relative slowness. His approach netted him a total 14,000 votes, just shy of the 17,800 votes collected by the Warmest 100 team.
The Warmest 100 website was spun up quickly as a relatively simple update from last year. The Hydra.js modular architecture library provides the basic structure, and Javascript drives the parallax scrolling effect.
It makes liberal use of cloud services, embedding players from SoundCloud and YouTube to play songs from the page itself, and traffic is measured using XiTi.com and Google Analytics. This year the team is using the CloudFlare Content Delivery Network (CDN) as a front-end to help manage load to the site.
We now have to wait for the countdown on 26 January to see how accurate this year’s predictions turn out to be.