The University of Technology Sydney has turned to artificial intelligence to model changing patterns of demand on the city’s train network to keep travellers safe during the pandemic.
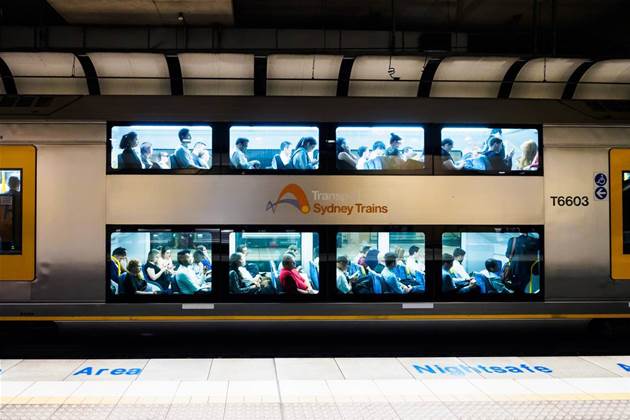
Distinguished Professor Fang Chen from the university’s Data Science Institute said her team looked at a variety of factors that contributed to the propagation of “primary delays” on one train to secondary delays that impact other services along the line or the whole network.
Speaking at the Intel AI Summit 2020, Chen said that in addition to the normal factors that cause delays such as weather, signal issues and hold-ups on the train platform (known as dwell time), the last few months had seen new factors emerge such as reduced capacity on trains and station platforms to curb the spread of the coronavirus that weren’t captured in Transport for NSW’s own delay models.
Data on traveller numbers was obtained from different sources such as the in-built weight sensors on Waratah trains, or AI analysis of CCTV on platforms.
Chen said that the size and complexity of rail networks meant that increased dwell time delays of only a few seconds resulting from a single socially distanced station and carriage could have a knock-on effect across the whole network.
“Rail operations are in an area where performance increasingly depends on availability to derive insights from complex data sets and make optimal decisions in real time,” she said.
Using a neural network, the team extracted multiple features from the data from a single day’s timetable including fleet type, throughput, occupancy time and runtime margin to train the model and produce an estimated on-time rate.
“Based on our experiment, our model can achieve more than 98 percent average accuracy on real-time data,” Chen said.
Combining this work with the AI video analysis for passenger throughput, which was also around 98 percent accurate compared to manual reporting of train occupancy, the team then began developing a model to improve dwell time at stations.
“We’re keen to explore whether we can systematically improve the dwell time of a platform and how it will impact the performance of the whole network if we can do so," Chen said.
“Even if the improved dwell time is only several seconds, it is still more important to have an accurate arrival and departure time.”
Data from the models can be used to more effectively manage traveller demand during the pandemic, where commuters have been urged to avoid travelling during peak hours to try and limit the spread of coronavirus.
Chen said the solution could also benefit the whole Sydney Trains network through better management of delays.
“It is difficult - almost impossible - to avoid the primary delays, but it is possible to consider how to reduce a primary delay so the impact for the whole network is less," she added.