NAB is using Europe’s “high-water benchmark” on data privacy as guardrails for its own expanding analytics ambitions, complicating its still-evolving data architecture.
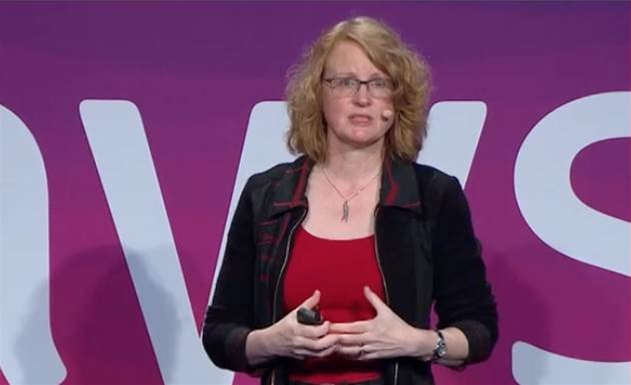
Chief data officer Glenda Crisp told the recent AWS Summit in Sydney that NAB is designing its data architecture to meet foreseeable higher standards of privacy in the domestic market.
The bank is already subject to the general data protection regulation (GDPR) rules since it processes data relating to European economic area (EEA) residents.
Crisp noted that the initiation of GDPR-like rights for consumers - such as the ‘right to be forgotten’ and the right to demand a human be inserted into an otherwise automated process - were complicated problems to architecturally resolve.
However, she indicated a desire to solve for them now - with a view to them potentially being adopted as part of future domestic privacy rules.
“On privacy, I think it's important to take a global view on this and think about what's coming, what are the trends that are occurring?” Crisp said.
“The high-water benchmark is in Europe with GDPR. Under GDPR, a person has the right to be forgotten. So we need to build our platform so that while we're enabling that right to be forgotten, we're not actually destroying or corrupting our downstream data models. This is not a simple thing to solve for.
“We also know that regulators are concerned about AI and machine learning. Again, going back to Europe and GDPR, an individual has the right to request a human be part of the decision.
“That means I [as a bank] can't create a locked-down straight-through process that's fully automated, so I can't go from machine learning model to decision engine to outcome. I need to build a way for a customer to request human intervention and have that human be part of the process in a seamless way.
“So we need to think about this as we build out our model deployment patterns.”
Crisp used her address at AWS Summit to lay out support for designing data architectures and analytics models to meet high standards of privacy and security while rooting out potential sources and causes of bias.
She noted current efforts involving the Australian Human Rights Commission pushing for the establishment of an AI Policy Council as evidence of broader community concerns “that exists across many industries around how data and AI is being used”.
“Everybody has to own this problem - we all need to step into this,” she said.
“We need to keep pushing for explainability and transparency in our models. We need to make sure we have the right governance in place to oversee how those models are created and how they continue to operate.”
Crisp said that NAB had well-established models for credit risk, market risk and liquidity risk, but that assurance and governance would need to be enhanced if and when part of these models were enhanced with AI or machine learning.
The bank said last year that its risk function was a target use case for machine learning. Other 'Big 4' banks like ANZ have also experimented with incorporating AI into certain risk functions.
“We, of course, are working very closely with our model risk management team and their model validation process,” Crisp said.
“We've had these processes for quite a while around credit risk, market risk, liquidity risk, and so we're uplifting those to include machine learning models.”
Data architecture evolves
NAB also used the summit to show off the past year of work in building out NAB’s data architecture.
In April last year, NAB announced its “first foray of data in the cloud” in the form of an elastic data lake built on AWS services.
It now has a series of key components that make up its data architecture.
The data lake - which is being called NAB data hub or NDH - is still there, though it is not an exclusively AWS affair.
“Informatica is the data management layer [in NDH] - that is our book-of-record for metadata, lineage, and data quality,” Crisp said.
“We use Kafka to stream in, we use Apache Beam to move data between the zones - between raw, curated and conformed - and we, of course, use S3 buckets [to store the data in].”
Crisp highlighted NAB’s thinking around organising its data and ensuring the lineage of the data - where it came from and how it ended up in the state it is in - is transparent.
“I tell my team on pretty much a daily basis, the difference between a [data] lake and a [data] swamp is metadata. If you have not tagged your data with both technical and business metadata, you've built a swamp and you actually don't know what data you have in there, and you certainly can't get the reuse opportunities out of it,” she said.
“Lineage is also important. It tells us not only where we got the data, but what we did to it: what calculations did we perform, what transformations occurred, did we aggregate the data, did we filter the data?
“All of that is really important to analytics team so that they know they got the right data for the right purpose.
“So at NAB, as we're building out our platform, we're actually landing data in our raw zone and tagging it with business and technical metadata, and then as we move it from raw to curated and [from] curated to conformed, we are publishing up those lineage components so that we have an automated trail of the lineage of the data as it moves through the cloud.”
From NDH, some of the uses of data include the previously-announced NAB discovery cloud as well as a newer element called NAB reporting services.
Discovery cloud is NAB’s “advanced analytics platform” and the workspace used by its data scientists.
While the data scientists are currently “huge users of Jupyter Notebooks” - a de facto standard open source tool in data science, Crisp noted other tools are being brought into the mix.
Notably, from an AWS standpoint, that includes SageMaker, an AWS managed service meant to help companies get machine learning algorithms trained and into production faster.
“We are piloting and POCing SageMaker and putting it through our security review process,” Crisp said.
Reporting services appears to be a relatively new element of the data architecture.
Crisp did not elaborate much on it, other than to say it is home to “our two newest tools, Thoughtspot and Power BI - also on the cloud.”
ThoughtSpot makes an AI-based analytics engine that promises to cut the time taken to find insights in data. Power BI is a fast-growing Microsoft service finding a home in more and more enterprises.
Crisp highlighted the evolution of the data environment, and in particular, the fact that it's not a pure AWS stack.
“The thing I would like you to take away from this architecture is yes, we use AWS and we [also] use open source and we use purchased software,” she said.
“We do this because we think it gives us the best mix and blend of capabilities that give our employees the tools they need to serve our customers.”
Crisp noted that the architectural diagram was over-simplified (“it looked way, way, way too busy”) and subject to change.
“This is as of today, and my team is extremely good at trying out new tech and techniques, and so this will look different in about six months' time,” she said.