NAB has found “hundreds” of use cases across the bank for machine learning and analytics technology and is in the process of standing them up.
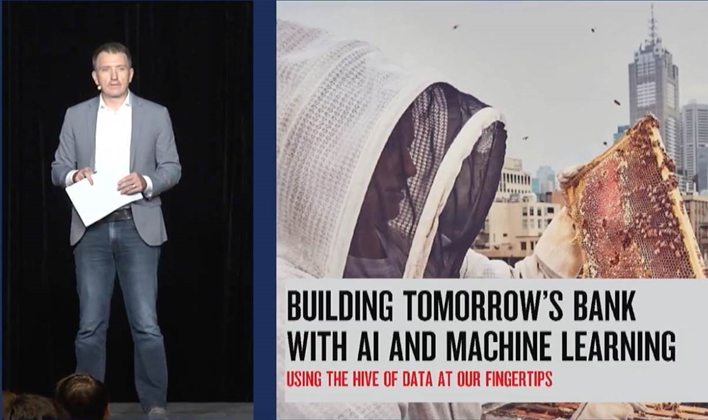
Speaking at the AWS summit in Singapore last month, executive general manager of the bank’s program management office, Kyle McNamara, provided extra detail on initiatives also spoken about at the AWS Sydney summit.
In Sydney, NAB revealed it is standing up an AWS-backed elastic data lake called discovery cloud, which will act as the foundation for its machine learning and analytics push.
In Singapore, however, NAB revealed the initial scope of its analytics efforts.
“At NAB there are literally hundreds of use cases in marketing, risk, operations and compliance that can and are starting to leverage analytics and machine learning,” McNamara said.
“We’re building analytics into our business strategy and building [out] all of these use cases.”
McNamara also provided extra detail on some of the early machine learning projects being pursued, such as the QuickBiz unsecured lending platform for small business.
Little was said on QuickBiz in Sydney, aside from it being held up as a case study for cloud-native product development at the bank, given it now decides one in every three small business loans.
McNamara said that QuickBiz can be used for unsecured loans of up to $100,000.
“Customers can choose a finance option that meets their needs, whether it’s a business loan, credit card or overdraft,” he said.
“The application is entirely online and only takes a couple of minutes to complete. And if you’re new to NAB you can provide a lot of ... your financial information directly from your accounting software like Xero or MYOB, and we’re able to do the analysis [on it] that way.”
McNamara said that adjudicating credit applications required the bank to take and consider a range of data such as “financial and behaviour attributes, your balance and payment schedules, the debt you owe, and your credit bureau file”.
QuickBiz still uses this data but also looks at the text detail of transaction records in order to score and determine the success or failure of credit applications.
“We started to concentrate on the transaction detail, specifically the text within the transaction and what we could learn from the text ... to help us identify the future potential for default,” McNamara said.
“That’s very much where machine learning comes in.
“Most importantly, using that information doesn’t require the customer to provide us any additional information - we have the data, it’s just a different way to look at it.”
McNamara said the development team behind QuickBiz used a variety of natural language processing techniques and machine learning algorithms to create the loan decision product.
“Ultimately we came up with the optimal solution which was a gradient-boosted model,” he said.
“We used a gradient-boosted model because it provided the best mix between the performance of the model to help us separate high risk customers from low risk customers, and the interpretability of the model.
“So in other words the model allowed us to understand why an application scored high or low, and also provided some additional predictive power which, at the end of the day, allowed us to approve more customers whilst still staying within the overall bank risk profile.”
McNamara said that QuickBiz took a total of 14 weeks to develop on top of the AWS stack.
He reiterated comments made by the bank’s chief technology and operations officer Patrick Wright in Sydney regarding the success of the project.
“It is one of the highest rated digital services based on the customer satisfaction scores that we take,” McNamara added.