Airbnb is overhauling the way data generated by users on its website is ingested into its Hive data warehouse to reduce outages and bring greater accuracy and reliability to its decision-making.
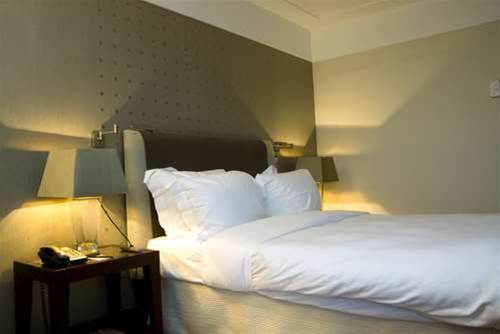
Data infrastructure software engineer Krishna Puttaswamy told the 2016 Hadoop Summit last week that the company needed to improve the timeliness, completeness and quality of its data reserves.
“Airbnb wants to offer the best travel experiences to our users and we believe data can play a critical role in offering those experiences,” Puttaswamy said.
Airbnb primarily drives insights out of its data through the use of machine learning models, though it also surfaces data via a set of dashboards.
“We have various products and various teams using machine learning models built on top of the data in the warehouse,” Puttaswamy said.
“We have a number of models to make sure our users are protected. For example, when a user logs in we have a model called 'account takeover' which analyses whether this particular user is a legitimate user or someone took over his or her account and is logging in on their behalf.
“Similarly, machine learning models are super useful for matching users to listings. We want to show listings that are most appropriate to users.
“We also want to take into account both the user’s and host’s interests so all of them are happy.”
The outage whiteboard
However, when Puttaswamy joined two years ago, he found “a lot of problems”.
“All the data we used to get was raw JSON [format] without any schemas,” Puttaswamy said.
“We had over 800 types of [data] events emitted from different sources, and these events were too fragile. It was too easy to break them, and breaking events and data pipelines in the warehouse was very common.
“Moreover we didn’t have proper monitoring on all the various systems we ran, which exacerbated the problem.
“The end result of this was there were too many outages, and many of the dashboards that we had were either missing data or the data quality wasn’t high, so it made a lot of our users lose trust on the data infrastructure.”
At the time, the data team used a whiteboard to track “the number of days since the last outage. For a while, it was always in single digits,” Puttaswamy said.
“The most important dashboards about bookings and user sign-ups used to be broken.”
Aside from feeding internal dashboards and machine learning models, Airbnb also uses data to judge how experimental tweaks to its website and services are tracking.
“Experimentation is huge,” Puttaswamy said.
“We have lots of events emitted from various experiments that we run, and we want to analyse that and make sure we give feedback to the developers on whether the experiment is doing well or not, and similarly when an experiment is launched we want to monitor the events quickly and give feedback quickly so if something is broken it doesn’t take days to detect all these problems.”
But this process, too, was broken.
“Once, it so happened that because of data quality issues the experiment analysis we did was all wrong,” Puttaswamy said.
The product manager then proceeded to email his team: “Hi team, This is partly a PSA to let you know ERF dashboard data hasn’t been up to date / accurate for several weeks now. Do not rely on the ERF dashboard for information about your experiment”.
“This was an actual email,” Puttaswamy said. “It was really bad.
“So we started looking through this system, trying to systematically analyse why it was so bad, and what we could do to make it better.”
The path to transformation
While on the surface bringing reliability to event data ingestion looked simple, it was in reality a “very hard problem”, Puttaswamy said.
Taken end-to-end, the data ingestion pipeline was “extremely complex” with a large number of components that were prone to “subtle” – undetectable - breakages.
Airbnb was – and still is – growing. It is constantly hiring developers who would change and add services, resulting in volatility.
“Since the data events were already fragile, the breakages were much more [frequent],” Puttaswamy said.
“But the biggest challenge was there is no ground truth.
“We didn’t know exactly how many events were emitted across all these different services. So because of this lack of ground truth in the warehouse we could not exactly tell how many messages were lost.
“And because there were no schemas, we didn’t know what a correct message looked like.”
Puttaswamy’s team embarked on a five-phase overhaul of its data ingestion system.
They started by hardening each individual component in the system, making sure it had appropriate monitoring and alerting.
“In the next phase we made sure that the end-to-end pipeline was solid,” Puttaswamy said.
The team then turned to data correctness – “defining schemas for the data events that are emitted and then making sure the schemas are enforced in all the right places.”
“Next, we built a system for anomaly detection that can catch not just big changes but even subtle changes in the data,” he said.
The final phase of change was to make the ingestion process more real-time, in part through the introduction of Spark Streaming technology.
“Until now, we ran [ingestion] in batch mode, which ran either at daily or sometimes hourly boundaries,” Puttaswamy said.
“We wanted to make it much more real-time.
“Initially we tried hours. Now we are doing [it] to a granularity of tens of minutes and our goal is to get to less than two minutes by the end of the year.”
Puttaswamy said the real-time engine is currently capable of ingesting “over five billion events every day with the loss of less than 100 events a day".
“Even when there is a loss we can exactly pinpoint which host lost the event and within a host, which particular process was responsible for losing the event,” he said.