Apache Hadoop is an open source technology that offers a radically cheaper alternative to the processing and storage of large amounts of unstructured data.
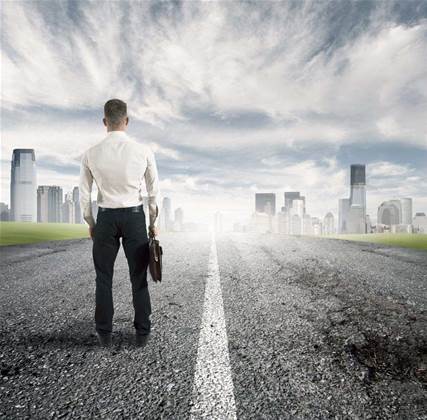
But for all of its potential benefits, Hadoop can be an uncomfortable fit within many IT environments. It challenges traditional approaches to data warehousing architecture, to the way in which IT projects are funded, and in some cases - can even threaten jobs.
On that basis, selling a Hadoop-based analytics project into a business is not as straightforward as a back-of-the-envelope cost calculation might suggest.
A different approach
Hadoop features characteristics that aren't shared with most data processing solutions IT departments have supported to date:
- the core software is 100 percent free and available for just about any form of use,
- the core software is entirely open source and can be downloaded directly off the internet,
- Hadoop can perform extremely well using low cost network equipment and does not require expensive high end server hardware, a high performance SAN or NAS, or tape or disk-based archival, and
- a properly configured Hadoop cluster can provide data protection without requiring additional tools.
The fundamental selling point of the technology is that doesn't require a large capital investment to get started.
Equally, however, some of these positive attributes have presented challenges for individuals pursuing big data projects inside large organisations.
Some CIOs are naturally cautious about adopting open source technologies without feeling confident adequate support structures are on the ground in Australia.
They are also likely to be cautious about any claim that Hadoop reduces dependence on RAID designs that have been a staple of data protection in the enterprise for decades.
And finally, Hadoop is radically different enough that some storage, server and data warehousing engineers will see a potential for the technology to obsolete their (vendor-specific) skills. As with any technology project, change management is crucial. We'll explore each of these barriers on page two.
Tip #1: Focus on business benefits
There is ample data available online to help sell the benefits of adopting new approaches to data management and analytics. A very healthy industry is being formed around big data and in particular Hadoop. You would be wise to make use of the massive body of work already published.
It's relatively easy to win an argument on the technical merits of the Hadoop architecture. Typically, the earliest business cases have been formed around:
- reducing data warehousing costs,
- faster time to market for decisions,
- validation for retaining and leveraging historical data, and
- leveraging 'sunk costs' of older hardware that would otherwise have been retired.
Ideally, however, these are supporting arguments for a project that needs to be more compelling.
The best business case for Hadoop is written by an author with a question in mind for which the business urgently requires an answer. And that answer should have the power to inform or validate a new approach to your organisation's way of doing business - something as critical as marketing or distribution strategies.
Tip #2: Start small
Even when you have a big question in mind to answer, Apache Hadoop offers a great opportunity to 'start small' and first win over your peers with a proof-of-concept which offers fast results at low cost.
Large organisations adopting Hadoop tend to have delivered proof of concepts using little more than the freely-available software running on commodity servers, before investing any dollars in supported distributions, dashboards and management tools on top of the base platform.
You may choose to first introduce Hadoop skills into the IT department for low-risk use cases such as:
- part of new dev and test platforms,
- to reduce the cost of retaining machine data and log files,
- as a temporary data store for Extract, Transform & Load (ETL) requirements
IT staff may relish the challenge of building a small Hadoop cluster using six to eight decommissioned desktop PC systems, on an old blade chassis with low power blades or on rack servers due for retirement.
We've also seen great success within large financial organisations hosting 'hack days', where new technologies are put in front of a mix of both IT staff, developers and business analysts, with an opportunity to learn about it, play with it, and dream up new possibilities for the business as a team.
We've also seen business cases approved without the use of any on-premise hardware - by pushing non-critical data onto Hadoop instances hosted on third party cloud platforms. The ability to spin up (and spin down) a virtual machine in minutes, without seeking external funding beyond your corporate expense account, is incredibly powerful.
You will, however, need to be cognisant of the fears many in your organisation would hold about pushing any corporate data onto a third party cloud. You will either need to go through the necessary approvals processes (which presents the unfortunate potential for your project to be scrapped before it begins) or make a very calculated gamble based on measurable results from non-critical data.
Also in Hadoop week:
In any case, it is advisable to keep pilots as simple as possible - senior IT execs are more likely to be won over by the speed of a result than by the magnitude of the problem you first attempt to solve.
Some of the most successful deployments of Hadoop have started from development or proof of concept clusters of a little as three or four desktop grade personal computers, a small desktop grade network switch, and an interesting data analytics challenge to solve.
Yahoo!, for example, started using Hadoop with just four servers in a development project. As of March 2013 they were reported to have over 42,000 servers storing and performing analysis on hundreds of petabytes of data.
Tip #3 - Communicate
When you take concepts around Hadoop (and big data generally) beyond your IT team, it's important to start with a clear common vocabulary for everyone to use when discussing your business case.
You could consider writing articles for your in-house newsletter and provide high-level introductions to key topics and examples of success stories in your industry. Few HR departments burdened with the challenge of publishing an in-house newsletter each month will say no to unsolicited articles from the IT department, provided you can make the content relevant and easy to consume. (We tend to find iTnews.com.au popping up on a lot of intranet sites for this very reason.)
You might consider running some educational workshops on data analytics prior to presenting your business case or proposal, being sure to engage with those stakeholders likely to be a hard sell further down the track.
Lunchtime 'brown bag' workshops with an open door 'walk in' format can bring about good results - just make sure you have a few backers in the room with you.
It is important to get the tone right - these sessions are about education. Nobody likes to hear a colleague come off sounding like a salesman. But most of us are open to being offered the opportunity to learn about something new if it is presented in an engaging and informative way.
Your sessions might cover topics like:
- What is 'big data' and why is it important?
- Case studies on competitors and peers sharing results with this technology
- What is open source and how it is licensed, distributed and supported?
Make sure you explain the topics in plain language anyone can understand. Avoid too much jargon or three letter acronyms. If you can explain the opportunity without having to wade knee deep in geek speak, you will find a lot less resistance.
Consider using analogies that make it easy to grasp potentially complex concepts. MapReduce is complex, and the technical overhead of breaking up and processing multiple 'chunks' of a data set isn't easy to describe. How would you explain it in a language the board can understand? How about this example:
Imagine trying to complete a head count of how many children are playing soccer on a large community sports field at 9am on a Saturday.
You could send one person out to count every single child on the field one at a time, which may take a long time, and most likely end up with errors as children moved around the field through the course of the morning.
But if you broke the problem up into lots of little bits by dispatching all of the team captains in parallel to count how many players they have in their team on the field at exactly 9AM, have them report back with a single number (sub-total) for their individual teams, then sum up those team counts and arrive at a final (grand total) number of children on the field at that time, it would take a lot less time, and provide a more accurate result at less effort. This is what Map Reduce does with data.
Read on for how to overcome common barriers to big data projects...
Many deeply entrenched ideas about how to address traditional enterprise data storage and analysis needs are directly challenged by Hadoop.
The first entrenched attitude you will need to dispel is that proposing open source is somehow a career-limiting move.
Open source and software support
Open source software is usually developed by a community of like-minded individuals, distributed freely online under known license mechanisms (Apache, GNU etc). The term open source refers to the fact that the source code can be downloaded and viewed or modified to suit any requirement.
The obvious benefit is avoiding upfront purchase and ongoing licensing costs, avoiding vendor lock-in and often (arguably) a higher quality of code due to the efforts of multiple, motivated individuals.
But some CIOs still perceive a range of technical and business risks associated with deploying open source in enterprise environments. They fear a lack of support or a 'throat to choke' when there are problems.
They fear that the software has been developed for back-room geeks rather than business users. They fear it is advocated for political reasons over practical ones. They fear its unpredictability, its potential to splinter.
IT professionals faced this same challenge when Linux made the transition from a pet project for computer geeks to a safe, secure, stable platform that powers the engine houses of the biggest names on the internet.
Linux initially seemed so radically different and it took years for some organisations to develop skills around it. Today many businesses or key technology platforms simply couldn’t live without it.
There is arguably enough movement around Hadoop to suggest that - like Linux - it will be supported as a vital tool in the enterprise IT armoury well into the future.
According to IDC, the Hadoop software market will be worth at least US$813 million (A$860 million) by 2016. IDC also predicts that the big data market will reach US$23 billion by 2016. While big data is unquestionably over-hyped, the dollars flowing into this sector should just about guarantee support well into the future.
CIOs should also take comfort from the distribution model for Hadoop - which again mirrors Linux.
Hadoop is available as a free download directly from the Apache Hadoop website. The Hadoop core is always remains under the open source Apache software license, such that any additional development or work on the Hadoop core must also be made freely available.
Hadoop is also available via value-add suppliers that distribute Hadoop bundled with proprietary management, monitoring, reporting or security tools, or as part of a larger analytics suite. Some of these companies are new to Australia (MapR, Hortonworks, Cloudera), but they have formed partnerships with many of the companies your organisation already contracts with (HP, Intel, EMC etc). The ecosystem is growing.
For support, IT organisations can again choose between a massive global community surrounding the open source core (online forums, wikis, email mailing lists, blogs and FAQ websites), or seek support from distributions, systems integrators and consulting professionals that specialise in the technology.
Skills might be scarce on the ground in Australia today, but more options are becoming available on a weekly basis.
The incumbents
Your organisation will most likely have an existing data warehouse platform and employ staff to directly support that platform. There is a tendency, whenever disruptive technology is introduced, for some of these individuals to search for reasons why Hadoop doesn't tick all the boxes.
You should argue that traditional architecture approaches to data warehousing do not need to compete with or conflict with your proposal to bring Hadoop into your organisation. Hadoop, as described in our FAQ section earlier this week, aims to derive results from large volumes of unstructured data and can happily live as a complementary solution to your existing data warehouse.
If you engage your existing database and analytics staff and share your vision with them from the outset, they are far likely to act against your interests. Reach out early and solicit their input and map out any issues, risks or concerns they might share.
Risks to data integrity
You may also come up against concerns that Hadoop plays fast and loose with data integrity, at least when compared with the RAID architectures used in most existing systems.
RAID refers to redundant arrays of independent or inexpensive disks to provide data protection and disk redundancy in case of hard drive failure. Enterprise storage platforms such as network attached storage (NAS) and storage area network (NAS) usually employ some form of RAID configuration.
The file system is a logical overlay spread across multiple physical hard drives, and data is written across multiple disks to provide disk redundancy. If one disk fails, copies of data is available on one or more other disks.
RAID doesn't tend to be applied within the Hadoop architecture. But data can be just as well if not better protected, it's simply a different approach.
Like any storage platform in traditional enterprise platforms, the Hadoop distributed file system (HDFS) storage platform requires careful planning to ensure data availability and integrity.
Files copied into a HDFS cluster are immediately broken up into chunks and distributed across multiple nodes in cluster. As files are loading into HDFS, Hadoop distributes these chunks to multiple nodes based on two key configuration settings: chunk size, in bytes (dfs.block.size) and replication factor (dfs.replication)
Chunk size is usually one of 64MB, 128MB, 256MB or 512MB. You would usually need to do a series of designs and tests to arrive at the ideal size based on input data size to be processed and the compute power of each node.
The replication factor sets the number of copies of each chunk to be spread across the cluster. By default, the replication factor is set to three - so each chunk will be available on three independent nodes across the cluster.
This replication is used to improve data redundancy and also distribute compute workloads into more manageable pieces.
So rather than writing data across multiple disks in a single server at a file system level the way RAID does, disks in a HDFS cluster are treated as as "just a bunch of disks" (JBOD) and data is written across multiple nodes across the cluster. If one disk fails in a node, the data is available from one or more other nodes across the cluster.
Have you deployed Hadoop in your organisation? What tips would you offer your peers on putting a business case forward?