The technology that powers your social networks, your favourite search engines, your online gaming experience and your anti-spam services is provocatively accessible to every enterprise, thanks to an open source project named after a child's stuffed toy.
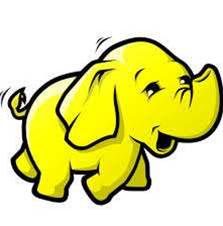
Apache Hadoop is the open source release of a technology that preceded just about every data storage and analytics tool that has since been labelled 'big data'.
It is - in many respects - the foundation of new types of services we've never seen before, and many businesses have quickly found themselves playing catch up for fear of being left behind. While we don't all have the unique technology requirements of a Google or a Facebook, any IT shop with terabytes of data streaming in from every direction will find it a useful tool.
Just about every organisation is being challenged to manage structured data from their traditional enterprise systems, as well as unstructured data from numerous other sources. We've become comfortable with managing many terabytes of structured data - given enough time to process it.
Big Data relates to comfortably storing and processing unstructured data in the order of multiple petabytes. Unstructured data might include, for example, the data generated from routers, firewalls, servers, business and consumer websites, shopping cart click streams, real-time sensor logs, high volume financial transition data. Unstructured data is usually messy, it's mostly real time, and often needs to be analysed on the fly.
So by definition, Big Data can be defined by the 'three V's': volume of data (size), velocity of data (speed), and variety of data (type). In simple terms, we're talking about large volumes of unstructured live data.
Managing the capture, collection, storage and analysis of this type of data presents a wide range of new and unique challenges that traditional data warehouse technologies were not designed to cope with.
Want to experience the power of Hadoop for yourself? Sign up for iTnews' interactive webinar series:
What is Hadoop?
Hadoop is an open source platform you have probably heard of, but most likely don't know much about.
In essence, it's an open source data storage and compute platform that allows for the processing of large volumes of data at far greater speed and much lower cost.
Hadoop makes it possible to quickly, easily and cost effectively build very large scale distributed data storage and data processing solutions using low cost servers and low cost networking hardware. It scales on a near linear basis, and there really isn't a lot of rocket science required to make it work.
You can string together cheap computers to build distributed storage and compute clusters capable of storing and processing very large-scale data sets.
You can start out small with a single system for development and testing. You could run Hadoop on your laptop, or on one or more virtual machines on your servers, or in the cloud for that matter.
The beauty of it is that from humble beginnings as small as a single node, Hadoop can scale to tens of thousands of systems, allowing you to keep up with almost any storage or data processing growth or capacity requirements as they arise at a price that won’t send you broke in the process.
Hadoop isn't only relevant to the web-scale data problems faced by the like of Twitter, LinkedIn, Facebook, Google, Amazon, PayPal or eBay.
Thanks to its release as a freely available open source tool, that same technology is now used on a daily basis by organisations of all sizes for analytics challenges once considered too hard or too expensive to run on traditional enterprise platforms.
Why is Hadoop so different?
The Hadoop platform is unique in its approach to both large-scale data storage and distributed data processing. Hadoop performs it's work on data on or near nodes actually storing the data you want to process, as opposed to copying that data across the cluster to a single node and then processing it.
Let's look at each of these in detail:
Hadoop Distributed File System (Storage)
In a Hadoop cluster, the Hadoop Distributed File System (HDFS) allows you to ingest data "into” Hadoop and then work as if your data is simultaneously on all of the disks and on all of the servers in your cluster. Essentially you treat it as if it's a single massive hard drive.
HDFS takes the total data copied into a Hadoop cluster, breaks it up into smaller chunks, and stores it in a distributed fashion across multiple servers, usually with three or more copies for redundancy.
A file in HDFS usually consists of many blocks (large blocks of 64MB and above) distributed across the cluster. The main components of HDFS are the NameNode, Secondary NameNode, and DataNodes.
The NameNode is the master of the HDFS system, and maintains the name space, as in directories and files. The NameNode manages data blocks stored on DataNodes.
The Secondary NameNode is responsible for regular data checkpoints, so that in the event of a NameNode failure of any kind, you can restart the HDFS NameNode using the latest checkpoint.
The DataNodes are the HDFS slaves deployed on every machine in the Hadoop cluster. DataNodes provide the actual storage, and are responsible for serving read and write requests.
In properly configured Hadoop clusters, if a server fails, copies of the "chunks" of data on that server are also stored on two or more other servers in the cluster. Hadoop automatically discovers when a server has failed, and immediately replicates copies of the data that was on the failed server across the cluster to ensure the minimum number of copies is maintained. Administrators can configure how many copies they want to store across the cluster.
By simply replacing a failed server with a "blank" new one, a Hadoop cluster immediately rebalances the distribution of data and compute workload to bring the new server back into the cluster.
MapReduce (processing)
Hadoop provides a new approach to distributed computing by implementing an idea called MapReduce, originally developed at Google.
MapReduce is essentially a programming model for processing massive data sets with a parallel distributed algorithm that allows fault tolerant clustering of commodity off the shelf (COTS) low cost computers. It allows for the splitting, processing and aggregation of large data sets.
MapReduce is unique in that it runs your applications either on the actual nodes where copies of the relevant data are hosted, or on nodes as close to the data as it can - in the same rack, if possible. This dramatically reduces the amount of copying of data across the network.
The main components of MapReduce are the JobTracker, TaskTrackers, and the JobHistoryServer.
The JobTracker is the master of the Hadoop processing system, and manages jobs and resources in the Hadoop cluster. The JobTracker schedules jobs as close to the actual data being processed as possible, ideally on a TaskTracker which is running on the same DataNode (server) as the underlying data block.
The TaskTrackers are slaves deployed onto each machine in a Hadoop cluster, and are responsible for running the map and reduce tasks as instructed by the JobTracker.
The JobHistoryServer serves historical information about completed applications. Typically the JobHistory server is deployed with JobTracker, but ideally it is run as a separate process.
A new era of data analytics
Hadoop has changed the landscape of data analytics in ways many industries are still coming to grips with.
US President Barack Obama's 2012 presidential campaign used Hadoop to predict in near real time where they needed to send teams of volunteers out on the streets and knocking on doors to focus their efforts to swing voters.
China Mobile uses Apache Hadoop - in combination with several supporting technologies - to serve billing inquiries for a staggering 650 million mobile phone customers.
A wider range of companies with asset management challenges (such as power utilities) are using Hadoop to ingest image and spatial data to map their power grids, poles, wires and surrounding vegetation.
Read on for Hadoop FAQ's - How is Hadoop distributed and supported? Where to next for Hadoop?