How is your maths today?
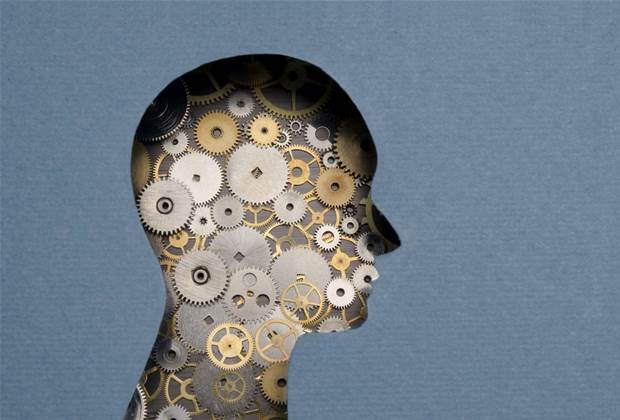
Let's assume you need to find out if you have liver cancer.
Assume the test for liver cancer gives false-positives (that is, the test is positive, but you don’t have cancer) 10 percent of the time, and false-negatives (the test is negative, but you do have cancer) 20 percent of the time, and the general risk of having liver cancer is one percent.
The test comes back positive. How likely is it that you actually do have liver cancer?
If you’re like most people, your answer will be staggeringly wrong, because humans are extremely bad at estimating risk using just their brains. The answer is you’re only 7.04 percent likely to have liver cancer. How close were you?
This is a very simple example. Most of the more important decisions we could make in our lives or careers involve many more variables, less certainty about the data, and are generally much messier. Does having a lot more data to work with help?
Monash University recently hosted a gathering of some of the leading users of so-called ‘big data’ at its Clayton campus in Melbourne. Attendees from the likes of Telstra, ANZ, Coles, the Department of Immigration and Border Protection and Walmart each shared how they use data analysis to help make decisions.
One attendee grandly declared that we are in “an age of evidence based decision making.”
Monash alumnus Suja Chandrasekaran, now global chief technology and data officer at Walmart, said the US retail chain used to reserve the term “big data” for datasets larger than 100TB, but have now abandoned the term altogether and simply call everything ‘data’.
In fact, Walmart are interested in more than just data they generate, but “all the data relevant to Walmart”, no matter the source.
There was a general consensus on the day that analytical decision making requires specialist skills.
Professor Geoff Webb, from the Faculty of Information Technology at Monash, told how he came to work with “one of Australia’s largest retailers” a few years ago. The retailer stored a year of purchase history data, he said, and wanted to find out “if someone buys lingerie, what else do they buy?”
They did some data mining, and discovered that people who buy lingerie also buy confectionery.
Emboldened, they went looking for more interesting correlations. People who buy women’s outerwear also buy confectionery. What about men? Yep, them too. In fact, 95 percent of all customers buy confectionery.
The organisation had successfully discovered something banal and obvious using expensive, complicated tools. “It was at that stage that they decided they needed some professional help,” said Prof. Webb.
“You can collect all the data, but unless you really know what questions you want to ask, it’s going to be pretty pointless,” said Greg Turner, program manager for multichannel at Coles.
Once you have the data, and people who know what they’re doing, “What they might predict,” said Prof. Webb, “is limited primarily by their imagination.”
The Department of Immigration and Border Protection primed their imaginations with a question, according to First Assistant Secretary Gavin McCairns: “How do you risk assess six million air arrivals annually?”
The department wanted to reduce this complexity down to a simple traffic light output of red, amber, or green. And they wanted to make those calculations in real time. It used the Open Source statistical language R to build a working prototype in just six weeks.
Walmart’s sophistication has extended to the point that the retailer is looking at machine learning systems to propose prices based on analysis. Again, the final price will depend on whether human beings agree with them.
Monash university researchers are testing a similar system to advise surgeons on the best locations for radiotherapy ‘pellets’ for treating prostate cancer. Humans still have the final say, but algorithms provide coldly rational advice.
Good people is one thing, but there was strong agreement from all speakers that organisational culture is an important part of deriving business value from data analysis.
“You must have a safe-to-fail culture," said Patrick Eltridge, Chief Information Officer at Telstra.
“You’ve got to get to the point where you understand that the experimentation is the work. It’s not something you do before you do a business case to run a project to do the work.”
Eltridge cautioned about expecting instant results from a sudden interest in data and analytics.
“You can’t just dive into this space and get all of this for free, all at once, because a bunch of executive sponsors have read a couple of books. It’s a build-up of capability and culture and practice and learning that takes time.”
Correction: Originally the example at the start of this piece posited a scenario in which false negatives occured 25 percent of the time. For the answer to be 7.5, that number should have been 20. Kudos to the readers that did their sums and proved us wrong!