We are entering an era where we need to reshape our thinking about the relationship between managers and machines.
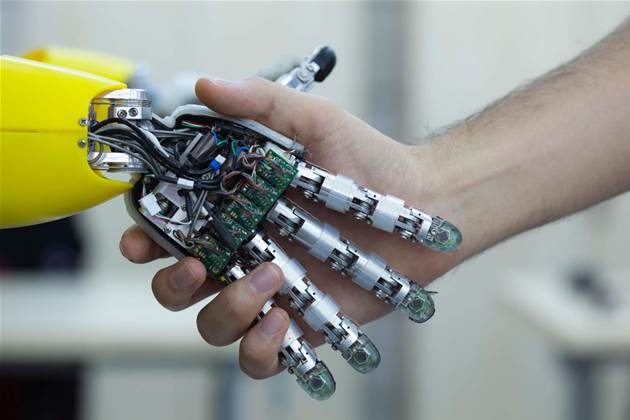
For those of us who have been in IT for some years, our earliest memory of computers was of glorified calculators.
I recall struggling with my statistics course at Sydney University in the 80’s, booking time in a laboratory to use some form of Basic to make a calculation. You would enter a simple command and through trial and error you were able to see if your program worked.
At the time it was commonly thought computers were are not able to think and required managers to routinely set the parameters for every calculation.
Today we are entering the era of machine learning – an era in which technology is able to provide many of the answers to our problems in reliable way.
We can now distribute a task – a question that needs answering - across 1000 processors, which each create a model answer. And at the end of that process, a consensus model is provided to predict the future.
Try doing that with your own grey matter! It’s enough to get you thinking, could machines make better managers than we are?
Machines, minds and cognitive load
Machines might indeed make better managers than people, on account of several key capabilities. There is one concept I’ve come across called cognitive load.
Cognitive load is all about how many items I can handle without impairing my judgement. It has been determined that the human mind has the capacity to store the equivalent of a million gigabytes of hard disk storage.
That’s compelling, but for most of us, we can usually only handle 6-7 items at a time. When we get to seven it starts to get more challenging – our judgment starts to become impaired.
I’d argue that most of us men are only capable of 5-6 things at a time as we all know that there is another item of Maslow’s Hierarchy that is always in our subconscious.
As CIOs, we pride ourselves on being great at multi-tasking. At any given point in time we are juggling business issues, future strategies, a operational IT hygiene concern along with staff matters, and on top of that all the programs and projects.
And we’re usually victims of our own success. The better we get at this, the more volume and velocity seems to come our way. The risk of impaired judgment increases.
Machines, on the other hand, don’t suffer from this same constraint. Could that mean they’ll one day be better at making managerial decisions?
These days we’re reading enough about the threat CMOs and CFOs are to our roles to bother thinking about the rise of the machines.
But we are starting to see some interesting movement in this space. McKinsey – the same group of management theorists that talked of computers as ‘dumb’ back in the 50’s - noted in August that a venture capital firm in Hong Kong (Deep Knowledge Ventures) has appointed a decision-making algorithm to its board of directors.
I guess I should be relieved Venturetec.Accelerator appointed me to its board and not some array of Violin Memory.
What is machine learning?
You may have heard of a reference to machine learning and dismissed this as AI or artificial intelligence (again). Well, yes it is AI but with a twist – this time it is all about systems that learn from data.
Unlike systems that follow logic and respond based on preset conditions, with machine learning we instead have the ability to learn from real time data. A simple example might be that temperature control in the smart building of the future will use the data from a myriad of sensors in the building to automatically adjust the heat.
I recall with fondness working in offices where this seasonality was pre-programmed and spring weather was anticipated. That never really worked.
More recently I came across a small $200 thermostat that has a 3.5-inch touch screen and is connected via wi-fi. Embedded inside is a sensor that you place in a room in your home, which sends the temperature in that part of the house back to the thermostat to help control the HVAC system.
So, when all your friends come over, this relatively uncomplicated piece of technology adjusts the temperature based on where and how many people are at home.
We tend to split machine learning into two camps: the supervised or the unsupervised. The supervised means the goal is given and the machine simply decides “on or off” as in the air conditioner being controlled by the remote thermostats.
However, in an unsupervised mode of machine learning, the patterns are themselves part of the learning. That’s where things are rapidly moving.
Machine learning and data mining
You can see some parallels in machine learning with what we already know about data mining. Machine learning is all about prediction from using data. It is the science of getting computers to act without being explicitly programmed that way. (There are no endless if then else logic loops that are in the code base).
Data mining is about the discovery of the ‘not known’ properties of data. It is about finding patterns in large data sets.
There will be a seismic shift in our industry when machine learning is as mainstream as data mining is today.
As a CIO, your role is to ensure that the possibilities enabled by machine learning are embraced by your organisation and not rejected as ‘just another technology widget’.
Which industries are at risk?
A 2013 report by Frey and Osborne – The Future of Employment [pdf] – gives us some insight into how susceptible a given job or industry is to computerisation. It predicted that 47 percent of the US workforce will be impacted by machine learning and robotics.
Not surprising, roles that require routine tasks are at risk, as such actions can be easily replicated by machines. But that’s not where machine learning comes in.
Management roles in areas like computer engineering are considered to be low risk. But roles in service, sales, office and admin support are all deemed high risk.
Looking at this study just 12 months on, through the lens of recent advances in machine learning, and I don’t see too many reasons why managerial roles can’t also be at risk.
Consider driverless cars. This is non-routine activity as the traffic conditions and weather patterns can vary, and current technology sensors have been reported to be less reliable when it rains. But with enough data coming in and processed at speed with machine learning, it is well on the way to being completely automated.
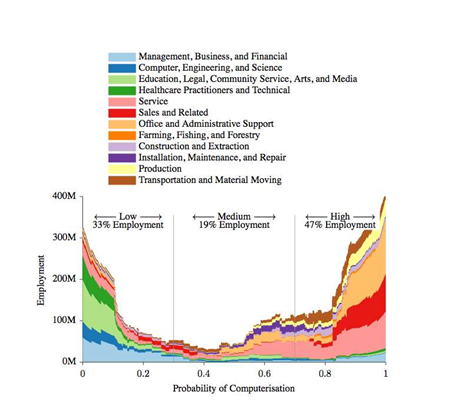
Source: Carl Frey & Michael Osborne 2013
While Frey and Osborne view IT as a lower risk of disruption, we can’t be complacent. Our industry won’t be free from restructuring.
At the same time, even the most bullish estimates view machine learning as still 4-5 years away from being prime time.
So what should a CIO be doing now? I would assume that machine learning and data mining are mega trends that certainly will cause disruption in the near future. I would assume that data mining will continue to be used to radically improve customer experience.
And if data mining is about providing ‘answers’, I would position myself to be the person best equipped to ask the right questions.
CIO or Chief Questioner?
As the CIO, you might not want to be known as the Chief Question Asker for fear of coming across like the great inquisitor.
As systems become more often hosted in the cloud and business decisions based on data and machine learning; the CIO can’t be in a position to have all of the answers, so much as we ever had them. There is much to be said for the CIO taking the more precarious position of not trying to be the person with the all of the ‘answers’.
We can also assumes machines will be better than we are at monitoring other machines, going back to the cognitive load discussion.
What is critical is that the CIO is taking a hard look at how their own role will evolve as well as those within their team and within their business partners.
The key will be to actively lead your organisation through this change.
If you are curious (and not sceptical) about machine learning, I recommend getting in front of the train and learning a little more. My suggestion is take one of the free online MOOC courses that exist on this subject.
Coursera’s MOOC on machine learning offers some incredible content.
If you are skeptical about machine learning, perhaps you should consider some practical examples of where it is already in use. The technology is already being used to manage card fraud.
Today we can train systems to throw up exceptions when a pattern of behavior changes, delegating a task to a human being to make a decision to accept or reject a credit card transaction.
To tease out the possibilities further, consider the evolution of real time payments at lighting fast speeds - how can a human keep up with the volume and velocity of these transactions? (It will have to be a machine.)
Further, the more I examine machine learning, the more it becomes apparent this is a new and emerging specialised capability that will be difficult to develop internally. It’s more than likely, sooner or later, going to be a component of your sourcing and partnering strategy.