Behind the scenes of Australian online jobs marketplace Airtasker sits Carl, busily working away to categorise the 80,000 tasks posted to the site in any given month.
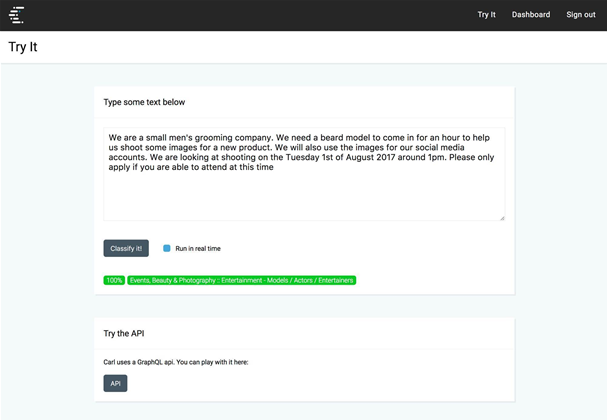
Carl is one of two machine learning tools the start-up has recently introduced to better automate its management of a sprawling collection of often wildly differing and random tasks.
Carl - built on the open source Scikit-learn machine learning library for Python - started life with a sample set of 200,000 tasks that a human input and manually categorised over several months.
Its learnings from that data - as well as the entire Wikipedia database that was later dropped into the sample set - have been applied to all live listings on the Airtasker site to sort tasks into around 140 groups.
At the moment Carl only runs in the background; despite having achieved a 95 percent confidence rating with its allocations so far, the company wants to build more confidence in the tool before setting it live for users.
Right now Airtasker users are presented with an open text field when they sign up to post their task so they’re not restricted to “rigid service categories”, Airtasker chief technology officer Paul Keen told iTnews.
“[We want to encourage users] to post tasks they would never think to outsource before. We found that our members started to get really creative beyond our wildest imagination of the types of tasks they were posting, and this is embedded into the magic of what Airtasker is,” Keen said.
Sorting tasks that range anywhere from ‘empty my fish tank’ to ‘queue up for an iPhone’ presented somewhat of an engineering challenge for Airtasker.
It initially experimented with machine learning-as-a-service tools but found that while these were quick with results, it was “hard to iterate and build upon your early success” - Keen says he was unable to get past an 80 percent confidence level.
While it ultimately chose to built the technology in-house, longer term Airtasker will likely adopt a hybrid approach, where specialised services like image recognition are sourced from AWS and Google tools, and more bespoke requirements are handled internally.
For now, Carl will continue to hone its learning in the background; at the moment it is being used to insure tasks and identify how Airtasker is performing in certain markets.
“We’re [also] currently building capabilities on type-ahead classification so, for example, if it’s a cleaning task you mention the number of rooms, or if it’s an end of lease clean you mention the date and price the task appropriately,” Keen said.
“The end goal is for tasks on the site to be well described, so that they can be accurately bid upon, and that that ultimately results in five star reviews.”
Alan the arbitrator
Alan is the second homegrown machine learning tool Airtasker has built.
Named after Alan Turing - the computer scientist who famously cracked the German Enigma code during WWII - the moderation tool is tasked with sifting through each week’s flood of new listings to catch out any dodgy requests.
“Alan learns how to pick out things like adult services, scams, and attempts to palm off school homework,” Keen said.
“We're building a machine to uncover users’ ulterior motives on Airtasker ... similarly to how Alan Turing built a machine to uncover ulterior motives during the war.”
Alan employs many of the same tactics a human would to identify listings that breach Airtasker’s guidelines; while keywords form a big part of its job, images, links and a poster’s history on the platform also have a role to play.
“Alan teaches himself which keywords, or items in photos are important. Some examples of words that are highly associated with breaking our rules include ‘sex’, ‘bank details’ and ‘homework’,” Keen said.
As posters increasingly figure out ways to get around Airtasker’s rules - for example by spelling out their phone number in a listing - Alan will need to match this pace with its own intelligence, and understanding context will become even more important.
“Most of the information that you would use to make [a moderation] decision would not come from the task, but would come from your past experiences, or information gathering processes,” Keen said.
“You know that "weeds" is ok, but "weed" is illegal because it's a type of drug. Alan learns this prior knowledge by reading through Wikipedia, or browsing through massive databases of images.”
Alan is “sitting low” at the moment while he learns as much as possible, Keen said, but is expected to become fully operational over the next new months.