eHealth NSW has begun a clinical pilot of a sepsis prediction system that uses Amazon SageMaker to detect the life-threatening condition in patients arriving at hospital emergency departments.
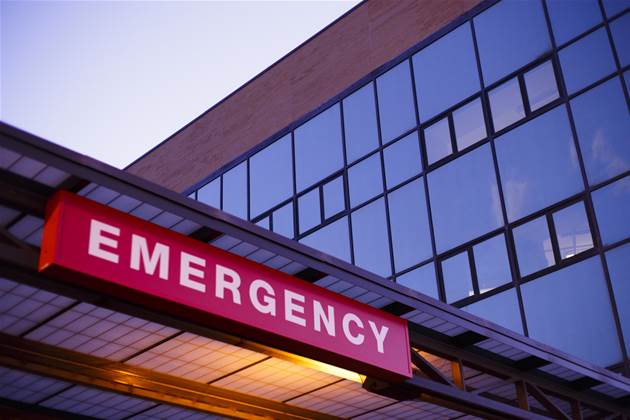
Senior data scientist at eHealth NSW, Mostafa Shaikh, told the AWS Sydney Summit last week that the project had recently reached “clinical go-live” at one unnamed hospital.
The pilot, which will run until August, is a precursor to a wider rollout if eHealth NSW, the digital arm of NSW Health, can demonstrate “significant improvements” in the early detection of sepsis, he said.
eHealth NSW has been working on a predictive sepsis detection system since at least 2019 to identify the people at risk of sepsis in emergency department (ED) waiting rooms from their vital signs.
Sepsis is a condition resulting from an infection that triggers an extreme immune system response, and can cause shock, organ failure or death.
Patients arriving at NSW hospitals with a possible septic condition currently have a 20 percent risk of death, a figure that climbs to 40 percent if not quickly treated with antibiotics.
“There are about 5000 deaths per year where the underlying primary cause is sepsis, and more than 15,000 patients get admitted to ICU ... due to septic shock or a severe septic condition,” Mostafa said.
While there is an existing rules-based sepsis detection system, this approach relies on a clinician filling out paper form, is “low sensitivity” and “often fails to flag true positive cases”.
“Since this is a paper-based approach, clinicians need to calculate the risk manually, which is often difficult to interact with the patient’s conditions and vital measures,” he said.
“From historical data, we have observed that the paper-based system could flag only two to two-and-a-half percent of the ED presentations as true positive, with a higher degree of false positives that often cause fatigue with ED nurses.
“But in reality, about five-to-seven percent of the presentations are sepsis-related. Therefore, there is room for improvement on the current rule-based system, which this project aims to address.”
Risk modelling
With the AI-based prediction system, eHealth NSW hopes to “increase the detection of true positive cases... and miss fewer cases” by “applying a clinical definition of sepsis in the model”.
The system uses a patient’s vitals – which are recorded directly into the state’s electronic medical record (eMR) system by the treating clinician – to calculate the risk of sepsis.
“As a patient arrives at the ED, usually a triage nurse consults with the patient, checking the patient’s vitals like heart rate, oxygen saturation, blood pressure and temperature,” Mostafa said.
“These observations are then recorded into the eMR system.
“The predictive sepsis system gets the data [that is] entered into the eMR in near real-time, and provides a list of patients who are at risk of sepsis waiting at the ED room.”
eHealth runs the vitals data through three different models; the accuracy of each model varies, depending on the age of the patient and other variables.
For instance, a linear model was found to work better for older patients, while a logistic regression model worked better for younger patients.
“Since there is no clear-cut winner in terms of these three models, we have taken all three models to score the same set of observations in the ensemble approach,” Mostafa said.
“The Amazon SageMaker model monitor system is helping us keep an eye on models’ performance metrics.”
Clinicians can access a list of patients predicted to have sepsis via a Tableau dashboard.
Mostafa said the dashboard works as a “blind spot detector to the clinicians for the patients who may be apparent looking healthy, but might develop a risk of sepsis if not addressed earlier”.
“Through this dashboard, clinicians can look at the most at risk patients and if required, double check their vitals and may apply earlier interventions like providing antibiotic or performing a blood gas test to reduce the risk of sepsis,” he said.
Next steps
eHealth NSW is measuring the success of the project using KPIs like reduced deaths, reduced time to treat with antibiotics and fewer re-admissions of patients who visited ED with a septic condition.
“A Bayesian structural time series model is being used to contrast the impact of the tool against similar sites without it, to account for any macro trends that are occurring,” Mostafa said.
Mostafa said eHealth NSW would look to “develop a business case to scale the solution to additional hospitals” if the clinical pilot demonstrated “significant improvements in patient outcomes”.