Atlassian has peeled back the architectural covers of a two-year-old, 500TB-plus internal data lake it has built known as Socrates.
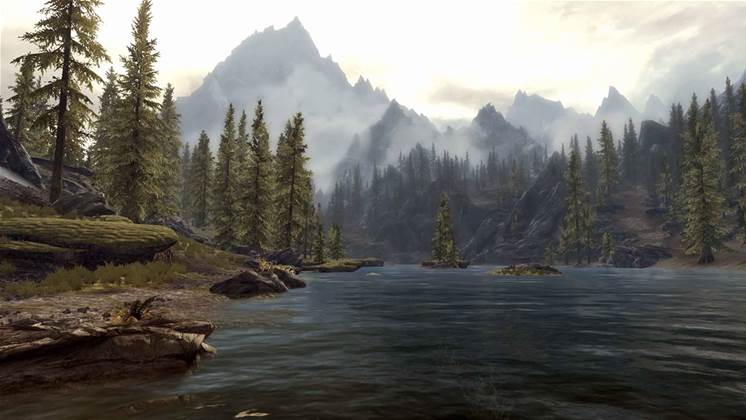
Speaking at AWS re:Invent 2017 in Las Vegas, Atlassian’s Sydney-based analytics platform manager Rohan Dhupelia showed off attempts to streamline portions of the data lake architecture by swapping out various components in favour of newer AWS services.
Though Socrates is still “pretty young”, it has already managed widespread adoption within Atlassian - and “has become pretty much the single source of analytics” for the company.
The name of the data lake is a hat-tip to the Socratic method, Dhupelia said: “a means of cooperative, argumentative dialogue between individuals, based on asking and answering questions to stimulate critical thinking”.
“We hope our platform allows users to do the same thing,” he said. “Essentially ask more questions to get down to the real deep answers.”
The data lake presently houses 500-plus terabytes of data in binary compressed format. Everything is stored in Amazon S3 buckets.
The lake ingests “over one billion events every day” and has about 1000 active users each week - equivalent to around half of all Atlassian employees.
Those users include internal analysts, data scientists and other data consumers across different departments and teams in the business.
The data lake is officially managed by Atlassian’s analytics platform team, led by Dhupelia, which is itself a subset of the company’s data engineering team. Dhupelia’s own team is a modest nine people.
However, the company is increasingly building out self-service capabilities to allow different businesses and teams to use the data lake as they wish - within certain boundaries - and to be able to query and analyse data without necessarily needing the help of a data engineer.
Work on building out Socrates is focused around four pillars: how data is ingested, prepared and organised, and then how enterprise users can discover and visualise it.
Ingesting data
When Atlassian first started work on its data lake in late 2015, Dhupelia said the aim was to create “a quick minimum viable product”.
“We really wanted to prove value fast,” he said.
The first iteration of the lake saw data being pulled in from the company’s web assets, its billing and CRM systems, and from ‘events’ created by its products.
“This essentially provided analysts visibility from the moment there was a click on a website to when a product was purchased and through to analytics around the behaviour of our users in-product as well,” Dhupelia said.
By early 2016, there had been an “explosion of microservices released across Atlassian”, which became additional sources of data.
“Socrates had also proven to be a very successful platform within the company [by this stage] with more people wanting to take advantage of its offerings,” Dhupelia said.
Data from all these different sources was being ingested into the data lake “by whatever means [was] necessary” but that began to cause issues as the data lake scaled.
“We tried to accommodate any means of integration but this led to .... a series of problems and it really took a toll on the team,” Dhupelia said.
“We had very brittle pipelines in that sourcing systems would change - either the data model or the API - or they would have downtime when we were trying to extract, and this was quite problematic for us.
“We began to see reliability issues with numerous extracts failing daily: up to 20-30 percent of them would fail every day.”
The pipelines also caused problems for the owners of the source systems.
“Our pull-based extracts would be a performance hit for them and would cause all sorts of failures and downtime on their side as well,” he said.
Dhupelia’s team also faced challenges understanding the architecture of the various source systems so they could connect into them and extract data.
By late 2016, there was a desire to re-architect ingestion. The answer came from a different internal team.
“Another Atlassian team released a platform called StreamHub which was an enterprise bus,” Dhupelia said.
“[Data] producers could essentially send data to a single endpoint and consumers could subscribe to events without having to worry about the complications of sourcing systems.
“We started to see our sourcing systems had already started to push events to StreamHub, and microservices and enterprise systems had started to subscribe to those events as well.
“So we saw this as a good opportunity for us to subscribe as well and take advantage of this vast amount of data that was available there.”
StreamHub is “essentially an event-driven architecture” and “schema registry … telling us how to read and materialise events in our data lake”. It is “mostly a microservices architecture built on EC2” and also incorporates AWS Kinesis for event streaming.
Actually landing data that passed through StreamHub in the data lake turned out to be a challenge.
The team wound up building a multi-stage “data landing framework” to get the data into a format where it could be exposed to data lake users. It is still working to streamline this process further.
Preparing data
Once ingested, the next thing Dhupelia’s team is interested in is how to help data consumers prepare and transform raw data for more sophisticated use cases.
“What we provide in the data lake is raw and unaltered data, but the true value comes from the prepared and transformed data,” he said.
“Things like dimensional models, aggregated and derived views that we can use to push on to reporting tools like Tableau or just make it easier for our users to query and understand data, and user-defined extracts that our users will use for machine learning models or for a particular analysis that they’re working on.”
Several challenges have emerged in the first two years of the lake’s existence.
One of those is how the company manages AWS EMR clusters that are used to run big data jobs for internal users.
“Cluster management is an issue for us,” Dhupelia said. “Particularly if you have a cluster running multiple jobs on it, it’s very difficult to attribute costs back to jobs and it’s also quite problematic to try to upgrade clusters.”
To resolve cluster management challenges, Dhupelia said his team shifted to a “job scoped clusters” model.
“This means that we dedicate one short-living cluster for every job and we shut it down essentially after the job is complete - so spin it up, run your job, shut it down,” he said.
“We use Airbnb’s open-sourced Airflow to manage the spin up and shut down of that.”
One of the key benefits of job scoped clusters is that it made chargeback simpler; Atlassian levies internal usage charges on its analytics resources.
“You can now understand the cost per entity or job and take advantage of things like per-second billing in EMR and you can also chargeback to your customers if you want,” he said.
“You can also upscale clusters for particular jobs that are more resource-intensive.”
Another data preparation problem to solve is what Dhupelia refers to as a “data engineering bottleneck”.
“Data analysts and data scientists and other data consumers are typically heavily reliant on data engineering for help to do things like create tables and [understand] how they run,” he said.
“We haven’t really solved the data engineering bottleneck yet. Users still need help creating aggregated and derived views [of the raw data], but to solve this we’ve created TaaS - transformation as a service.”
TaaS consists of a UI where users can specify and schedule transformations of raw data.
It is still very new - inside its first three months of availability - but Dhupelia was buoyed by its early success and potential.
Organising data in Socrates
A third pillar of work has focused on structuring and securing the data in Socrates, and who has access to it.
“This was pretty easy to do in an RDBMS [relational database management system] world but it’s proving to be a lot harder in a big data world,” Dhupelia said.
One of the early challenges was structuring the data lake in a way that would enable it to scale.
To do this, Atlassian has effectively divided the lake into four ‘areas’:
- Landed - this is not exposed to users or query engines. “We keep it around just in case we need to replay any ETL,” Dhupelia said.
- Raw - which consists of “partitioned and optimised data” that is exposed to users in the data lake. “We mask any sensitive data here as well,” he said.
- Modeled - this comprises “certified and conformed entities, [which are] typically entities that have been built and maintained by the data engineering team, and … contain critical business metrics or core referential data which is used by many users across the company.”
- Self-service - a space where teams can “build their own entities, upload their own data, [or] perform a transformation on some of the raw data that we’ve provided,” Dhupelia said.
The self-service capability was in part borne out of a desire by teams to be able “to structure their work in a way that’s meaningful for them,” Dhupelia said.
“They don’t want us to dictate how entities are named or how they should structure their spaces.”
The self-service part of the data lake is managed through Atlassian’s own IT service desk, which is underpinned by the company’s own Jira service desk product.
While teams can use it to request their own schema - essentially how they want to construct their little slice of big data - Dhupelia said his team “act as a bit of a gatekeeper” to prevent too many schemas proliferating in the data lake.
“We try to limit them to being at a team level or departmental level at most,” he said.
“When a user requests a self-service schema, a few things happen. One is we provision an S3 bucket for them and we tag it to the user and their business unit as well so we can charge it back.
“We then create an Active Directory group whereby they can maintain control and access to their buckets themselves. And we use Vault - essentially an open source tool designed to manage secrets - to control access rights.”
There are no limits placed on what a user can do with their self-service ‘zone’. “They can use it for whatever they want really,” Dhupelia said.
Discovering the data within
The final pillar of Socrates is also “probably the most neglected pillar in our data lake so far”, Dhupelia noted.
“Up until recent months, we believed that the other three pillars were of higher criticality before we could really look at this,” he said.
“But here we try to enable our users to find what they need, understand it and to be able to deep dive and find insights without having to interact with the data engineering team.”
Atlassian has come to the conclusion that finding data in its lake is hard. It is in the process of building search functionality on top of its data portal “to make it easier for others in the community to find data better”.
The search capability is still a work in progress, and Dhupelia is hoping to count on a community of data analysts and data scientists internally to contribute to the effort.
The company supports four main visualisation tools: Tableau, R Shiny, Zeppelin Notebooks and Redash, though it is flexible in supporting other tools outside of those.
“Teams want options,” Dhupelia said.
“Different tools have different pros and cons and we need to provide as much flexibility as we can here.”
However, the main way that Atlassian’s analysts interact with Socrates is using query engines.
It is in the process of shifting the way it does this, from running the open source query engine Presto itself to using Amazon’s Athena (which itself is based on an implementation of Presto).
“At the beginning of this year we decided to stop using Presto and move to [Amazon] Athena,” Dhupelia said.
The move has had a few bumps, not least because Atlassian is one of Athena’s early adopters.
“We started migrating to Athena as soon as it went GA [general availability] and we hit a number of hurdles and teething issues in the service along the way,” Dhupelia said.
One of the problems has been a lack of parity in functions and features compared to running Presto themselves.
“They are both running on the same tech but there were still some functions and features that weren’t working in Athena but worked in Presto, so we had to keep Presto up and running for a period of time while we had Athena up,” Dhupelia said.
Atlassian had also seen a couple of “surprisingly large bills” for its Athena usage, due to problems with monitoring tools.
“We haven’t really been paying attention for a while and then the end of the month comes and we get this surprisingly large bill,” Dhupelia said.
“It’s usually just because a user’s been querying it at too-high a frequency and there’s a bit of monitoring lacking at the moment. I’m sure it will improve in time.
“Overall we feel at the moment the benefits outweigh the costs and challenges, and Athena’s likely to improve and surpass probably anything that we can do with Presto.”
Incorporating more AWS services
Dhupelia said that as portions of the data lake’s underpinning technologies and components became commoditised, it made sense to swap them out for equivalent AWS services.
“For example [using] Kinesis versus running your own Kafka cluster, or Athena versus running your own Presto EMR cluster,” he said.
“This has helped us spend less time maintaining things and more time focusing on adding value elsewhere.”
However, he warned that spinning up a data lake was not as simple as buying AWS services.
“You can’t just spin up a Kinesis stream, a firehose, and have an instant data lake,” he said.
“You often have to build services around these things to make them work for you.”