For a brief period in the middle of the last decade, data scientists were the business world's most in-demand skill. The deliciously toxic mix of scarcity and demand drove salaries into the stratosphere. In some sectors — such as social media marketing — the annual pay rates exceeded $200k.
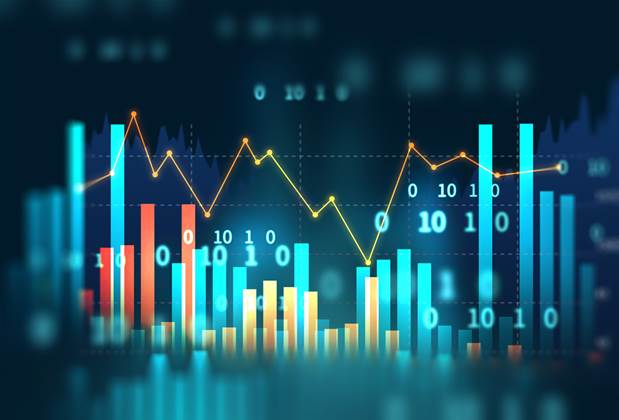
Often the organisations hiring these high priced data-alchemists had no idea what to do with them when they arrived.
Since that time technology, such as AWS's Amazon SageMaker, helped address the problem partly by automating a lot of the grunt work that kept the data scientists from doing their real job — running experiments.
So did a greater investment by the education sector in post-grad analytics.
The market while still hot like all technical skills is now only awful within normal parameters and these days, data scientists play a key role in driving data-driven cultures in business and empowering leaders to make evidence-based business decisions.
While historically the data science field has been relegated to the sphere of specialists, nowadays business leaders across all c-suite and management functions must have a sufficient level of data and analytics proficiency. And data science specialists must have effective communication skills in order to bring the leadership team along on the data story-telling journey.
Dr Alex Antic, managing director of the eponymous human-centred data science and AI consulting group, Dr Alex Antic Group defines the role of a data scientist as central in responding to core business challenges.
“The role of the data scientists, the data analytics professional, AI engineer is to solve some specific, hopefully, well-defined business problems, and to find the most suitable technological solutions to actually solve those problems,” said Antic.
“That means a deep understanding of the data that governs the business and trying to determine what's the simplest and easiest solution to develop to begin with, such that you can interrogate and validate the results, and then hopefully explain your findings to your colleagues, to your boss, to the broader customer base that you're trying to develop these solutions for.”
Anna Russell, data scientist and director at Polynomial Solutions said that the two most important skills for a data scientist are the ability to effectively frame the question that reflects the exact problem that the business is trying to solve, and then the soft skills to communicate in layman’s terms the results to business leaders.
According to Dr Catherine Lopes, founder and director at OpsDo Analytics, bridging skills are critical in translating the business problem into an analytical solution. And delivery skills are necessary for converting the solution into value for the business.
“A lot of data analytics work has been done in the process or in the matter of 'doing the doing', but not necessarily actually delivering. It doesn't matter if you're part of the data engineering, or you’re part of the data architect or you're part of the data visualisation or business analyst. You have the skills, but you have to convert this outcome into an actionable item that can be consumed on the value chain,” said Lopes.
According to Jamie Leach, chapter lead ANZ at FDATA A&NZ, data scientists should be able to produce visualisations of the key results in a way that boards and leadership teams can engage with.
“A data scientist is never going to cough up 10,000 lines in a table and hand that to the CEO and go, 'This is what the product's doing’, or ‘This is what the service is doing’. Any normal person, your eyes would blur, you wouldn't understand it,” said Leach.
“But if it's depicted in a visual way, some sort of chart, some sort of infographic, whatever that looks like, it is the c-suite’s job to make sure that they can understand it, get clarity out of the data. And if it's not being depicted or formatted in a way that is relatable to whatever the end goal is, they need to be able to go back to that data scientist and say, ‘We’re still not getting what you're trying to convey'.”
Despite the fact that leadership teams globally are upskilling to more effectively interrogate data, Macquarie University’s deputy dean, education and employability, Yvonne Breyer highlights the knowledge gap that still exists between data scientists and business leaders.
In order to breach this divide, Breyer stressed the importance of data storytelling.
“We have to help people to be in a position to simplify very complex matters and very complex issues that are coming from a number of directions and to cut through the fog and help the next person who is probably not an expert in a particular area, finance, for example, to get to the heart of the issue that they're trying to communicate,” said Breyer.
“Oftentimes when we code things in a story, it's much easier for those that aren't an expert in your area to follow and to actually discern, ‘What are the key points that you are trying to highlight and what do I need to direct my attention to?’”
While in the past it may have been the remit of the chief data officer, the chief analytics officer or the chief information officer to be data literate, according to Deloitte’s director of data analytics and AI, Angela Kim, all c-suite leaders including chief revenue officer, chief compliance officer or even chief marketing officer must be “data fluent”.
“As a c-suite leader, it's almost impossible to talk about your strategy without data,” said Kim.
“Leaders should really familiarise and educate themselves and learn about what technology, data and AI mean for your role and for the organisation so that you can make the most out of it so that you can really utilise it.”
Automation
Automation tools such as Amazon SageMaker and Azure’s Machine Learning Studio, have changed the nature of the data science field and the role of data scientists.
According to Antic, off the shelf tools have automated mundane tasks, allowing data scientist capability to be diverted to solve more challenging problems that require human input.
“It's also reduced human error in many cases where you have humans doing a lot of repetitive, boring tasks effectively, where error can easily creep in, setting a greater degree of validity and credibility over some of those tasks and end-results has really changed,” said Antic.
Digital Nation Australia spoke to Rada Stanic, the principal solutions architect at Amazon Web Services.
“What the data scientists are looking for is they want to increase their productivity. So they want to spend more time on doing interesting things rather than preparing data, yet, data preparation is an essential part of what they do,” she said.
According to Stanic, SageMaker and Data Wrangler are two tools that create efficiencies for data scientists, reducing their time spent on time-consuming tasks such as data preparation from weeks to minutes.
Stanic describes international racing organisation, Formula 1’s experience using SageMaker: “Imagine a company of that size, a business of that size, having 65 years’ worth of historic data and being able to bring that to life, to transform the experience of races and also the audience. And Formula 1 is able to give these near real time insights to the audiences based on effective use of this historic data and SageMaker trained machine learning models.”
While automation tools, when used effectively, can increase efficiency and productivity, Leach stressed the importance of good data governance to get the most out of the tools.
“If you have terrible data going in, you're never going to solve what you're trying to just by employing even the most expensive technology suite,” said Leach.
“We need to make sure we've got the right data going in and it's of the highest possible quality. And then we need to be crystal clear, what is the end goal? Because otherwise, you can just invest in the most amazing technology and still not achieve the baseline outcome that you want.”
Polynomial Solutions’ Russell said that for businesses with data scientists that are looking to reduce their workload, automation will be a helpful tool, but cautions against using them without the right skill sets.
“They can be dangerous if you get someone who isn't necessarily trained in machine learning and what the principles are of these techniques, he just goes in and starts building models and then commercialising them without really looking under the hood as to what they've built, that can be dangerous because it can give you false information.”