Google today released a set of code for its SyntaxNet natural language parser for neural networks as open source on the Github repository.
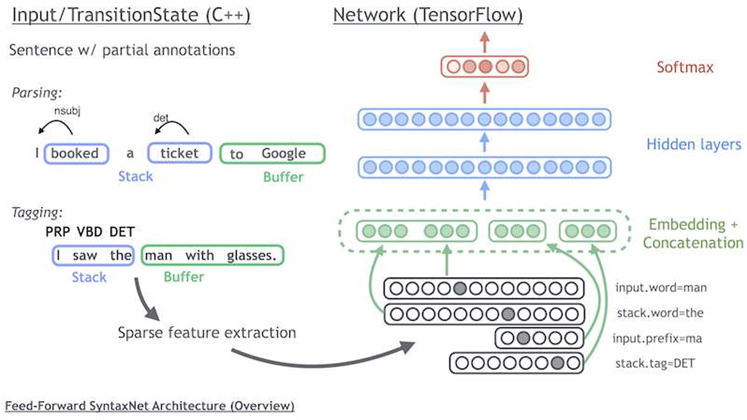
The models include the ready-trained English language Parsey McParseface parser, which can explain the function of each word in a given sentence, according to Slav Petrov, Google staff research scientist.
The moniker Parsey McParseface is a reference to an online campaign to name a British polar research ship Boaty McBoatface, which despite massive backing was ultimately unsuccessful.
SyntaxNet aims to provide developers and scientists with the tools to analyse linguistic structures of languages to explain the functional role of each word in sentences, Petrov said.
Natural language understanding is traditionally very difficult to achieve with computers.
This is due to ambiguities in human languages, which result in sentences of moderate lengths of 20 to 30 words having at times up to tens of thousands of different syntactic structures.
However, Google believes its SyntaxNet parser is the most accurate one in the world.
Petrov said that on well-formed text such as English newswire sentences, Parsey McParseface "recovers individual dependencies between words with over 94 percent accuracy".
This approaches human performance - linguists performing the same task reach 96 to 97 percent acccuracy, according to Petrov.
Parsey McParseface falls apart, however, when it comes to analysing sentences sourced from the web, managing only 90 percent accuracy on datasets. Even so, Petrov believes the accuracy rate is high enough to be useful in many applications.
SyntaxNet and the Parsey McParseface tool were released under the Apache 2.0 open source licence, and are implemented in Google's TensorFlow machine learning library.