The Australian Defence Force Academy (ADFA) is investigating intrusion detection and biocryptographic techniques to address gaps in policy-based network security.
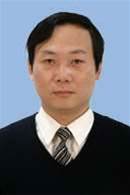
Although the technologies may come at a “very high cost”, according to ADFA professor Dr Jiankun Hu, they may be necessary to improve firewall-based network security, where “penetration is always possible”.
Hu told the Australian Computer Society’s national conference last week that most firewalls were vulnerable to targeted attacks.
One such attack cost General Motors Holden $6 million in car production in 2005, when it was forced to shut down its vehicle assembly plant in Adelaide for several hours after being infiltrated by what was thought to be the Zotob virus, he said.
“Firewalls are very easy to penetrate because the current firewall technology is based on a certain policy on access,” Hu told the conference.
“With a new virus coming in, or a new variation of attacks, those policies often cannot anticipate features of the new attack.
“So how can you prevent it? It’s impossible for a firewall. So penetration is always possible.”
Access control
Hu said network security had inherited a fundamental flaw of conventional cryptography, in that conventional, knowledge- or token-based methods could not be completely trusted to lock unauthorised users out.
“PIN and password indicates what you know and what you possess,” he said. “They do not tell you who you are and what you are. Who is presenting the tokens? That’s the fundamental problem.”
Hu suggested that biometrics such as fingerprint, face and iris patterns could improve identity detection, especially when used in conjunction with smartcards.
Research groups at ADFA were developing “fuzzy vaults” and “fuzzy extractors” to extract biometric information for use in encryption, he said.
Although attackers have fooled biometric scanners with photos of fingerprint, face or iris patterns in the past, Hu said “multi-modal biometrics” improved reliability by requiring multiple biometric identifiers.
“Liveliness detection” techniques could also determine if patterns belonged to a living person by using two LEDs with peak emissions at 530 and 640nm to detect certain characteristics of live fingers, he said.
Better intrusion detection systems
Hu warned that traditional intrusion detection systems were another area of weakness for many enterprise networks because they often failed to cope with traffic increases across the network.
"Anomaly intrusion detection" systems that monitored networks for abnormal behaviour were more scalable, he said, but had high false positive rates and could be fooled by carefully crafted attacks.
To address the issue of false positives, Hu has been researching the application of Hidden Markov Model (HMM) machine learning techniques.
The technique reduced false positive alarms by 48 percent in an experiment, compared with the single normal database detection scheme, Hu reported in the Journal of Network and Computer Applications in November 2009.
Although both biometric and new machine learning techniques came at a “very high cost”, Hu said organisations would find it cheaper to introduce the technologies than tolerate strategic or financial loss due to compromised systems.
He urged organisations involved in major infrastructure, national security or manufacturing in particular to consider the new approaches.
“The problem is that a lot of enterprises are not aware of these new generation systems or they are not keen to invest in them,” he said.
“But once they make their loss they have a problem. They seem to just want another firewall without realizing it is fundamentally penetrable sooner or later.”