In order to effectively mitigate AI risk, a systematic approach of identification and prioritisation is required, according to an article by McKinsey Research.
The authors; Kevin Buehler, Rachel Dooley, Liz Grennan, and Alex Singla of the article titled “Getting to know—and manage—your biggest AI risks”, believe that while significant value can be generated with AI, the technology could expose them to a rapidly evolving world of risks and ethical pitfalls.
McKinsey’s latest AI survey revealed that respondents getting the most value from AI were more likely than others to recognise and mitigate the risks that the technology presents.
“Organisations must put business-minded legal and risk-management teams alongside the data-science team at the centre of the AI development process,” the authors say.
“Waiting until after the development of AI models to determine where and how to mitigate risks is too inefficient and time consuming in a world of rapid AI deployments. Instead, risk analysis should be part of the initial AI model design, including the data collection and governance processes.”
By installing an informed risk identification and prioritisation plan, a more dynamic and effective safeguard against such threats can be created.
Beginning with the identification step, the authors suggest mapping risk categories against possible business contexts.
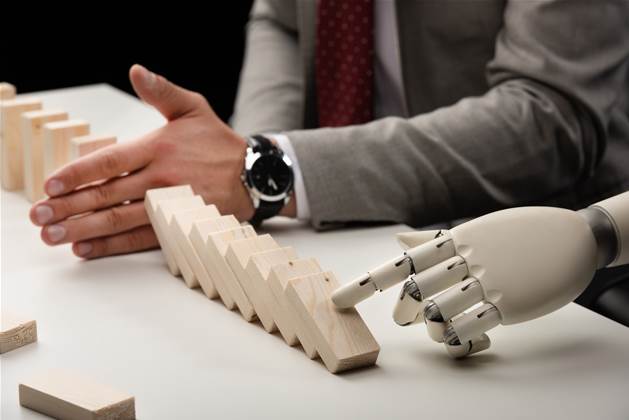
The six overarching types of AI risks listed include:
• Privacy
• Security
• Fairness
• Transparency
• Safety and performance
• Third party risks
“In our experience, most AI risks map to at least one of the overarching risk types just described, and they often span multiple types,” the authors say.
“Pinpointing the context in which these risks can occur can help provide guidance as to where mitigation measures should be directed.”
The six contexts identified include:
• Data
• Model selection and training
• Deployment and infrastructure
• Contracts and insurance
• Legal and regulatory
• Organisation and culture
After clearly defining and cataloguing the risks, assessing the list for the most significant risks and sequencing for mitigation is next.
“By prioritising the risks most likely to generate harm, organisations can help prevent AI liabilities from arising—and mitigate them quickly if they do,” the authors say.
“A strong methodology for such sequencing allows AI practitioners and legal personnel to triage AI use cases so they can focus their often-limited resources on those meriting the most attention.”
The authors acknowledge that best practice surrounding risk mitigation in this field is continually evolving, however they detail two methods of enabling the cataloguing and weighting of AI risk.
First, standard practices and model documentation allow for standardised policies in and clear standards for model development.
Secondly, independent review as well as systematic internal and external audits can help reinforce an organisation's AI risk mitigation.
“Based on recent headlines alone, it’s clear that global efforts to manage the risks of AI are just beginning,” the authors say.
“Therefore, the earlier organisations adopt concrete, dynamic frameworks to manage AI risks, the more successful their long-term AI efforts will be in creating value without material erosion.”